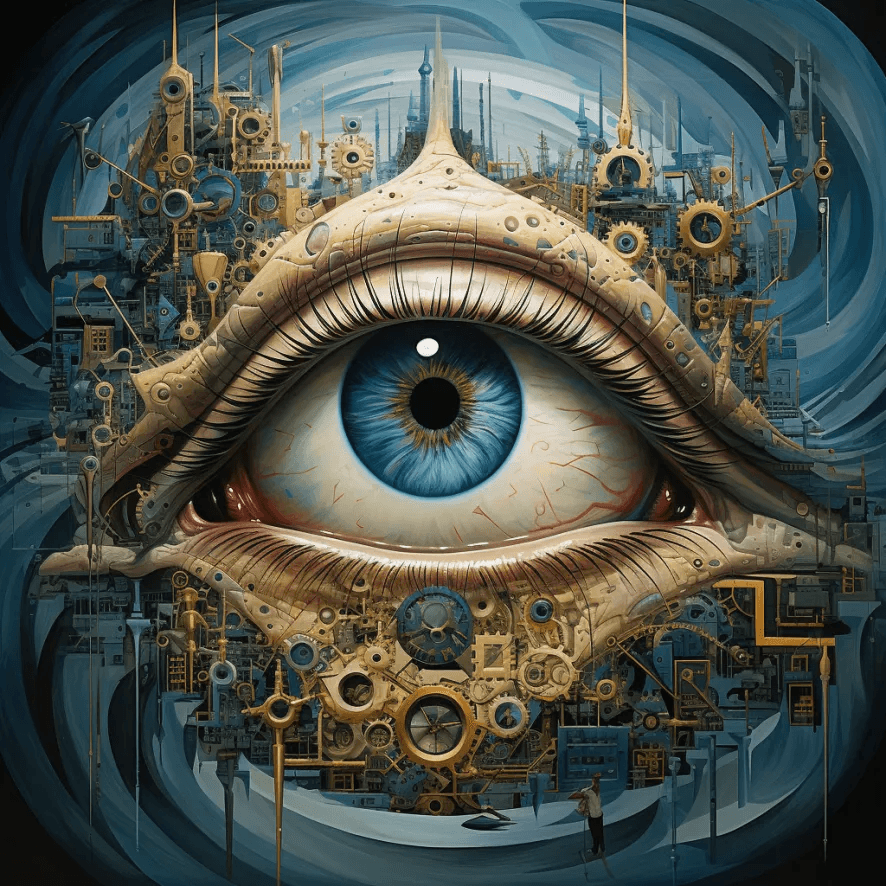
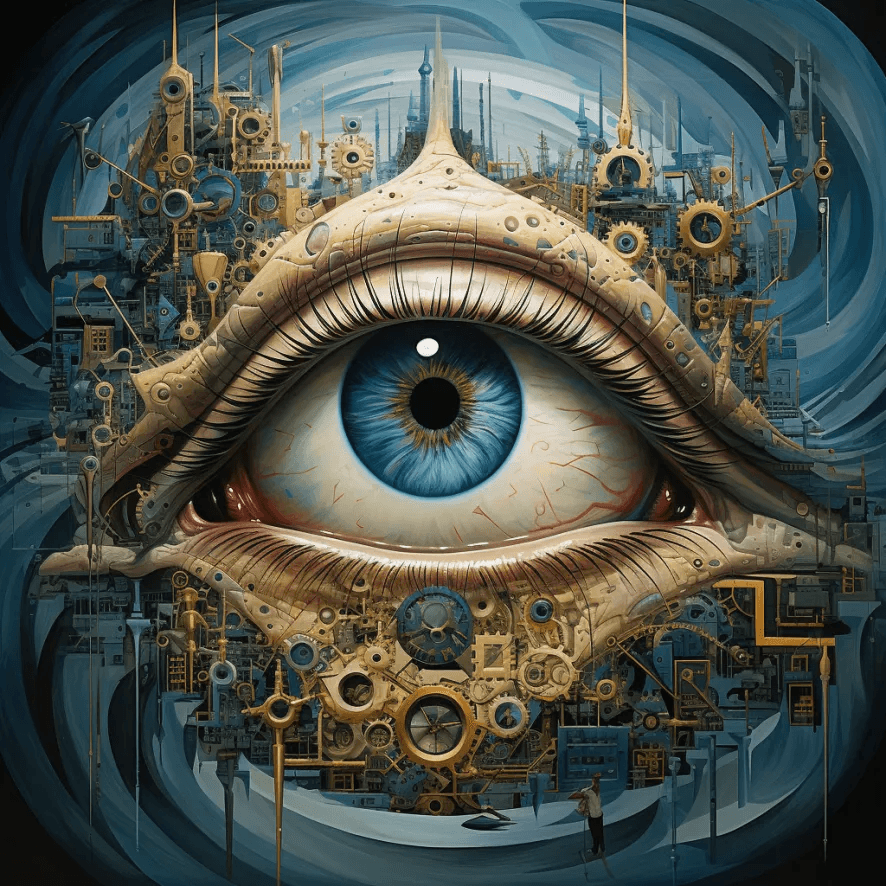
Epistemological Vigilance for Alignment
Epistemological Vigilance for Alignment
Epistemological Vigilance for Alignment
Adam Shimi
Jun 6, 2022
Nothing hampers Science and Engineering like unchecked assumptions.
As a concrete example of a field ridden with hidden premises, let's look at sociology. Sociologist must deal with the feedback of their object of study (people in social situations), their own social background, as well as the myriad of folk sociology notions floating in the memesphere. You might think that randomized surveys and statistics give you objective knowledge of the sociological world, but these tools also come with underlying assumptions — that the phenomenon under study must not depend on the fine structure of the social network, for example. In general, if you don’t realize this, you will then confidently misinterpret the results without considering the biases of your approach — as in asking kids to sort their play activities into three categories you defined in advance, and then seeing this as a “validation” of the classification.
How to avoid these mistakes? Epistemological vigilance, answer Pierre Bourdieu, Jean-Claude Chamboredon, and Jean-Claude Passeron in "Le métier de sociologue". They borrow the term from French philosopher of science Gaston Bachelard, to capture the attitude of always expliciting and questioning the assumptions behind notions, theories, models, experiments. So the naive sociologists err because they fail to maintain the restless epistemological vigilance that their field requires.
Alignment, like sociology, demands a perpetual questioning of unconscious assumptions. It’s because the alignment problem, and the way we know about it, goes against some of our most secure, obvious, and basic principles about knowledge and problem-solving. Thus we need a constant vigilance to keep them from sprouting again unnoticed and steering our work away from alignment.
In this post I thus make explicit these assumptions, and discuss why we have to be epistemologically vigilant about them.[1] Taken separately, none of these call to vigilance is specific to alignment — other fields fostered it first. What makes alignment unique is the combined undermining of all these assumptions together. Alignment researchers just can't avoid the epistemological struggle.
Here is my current list:[2]
Boundedness: the parameters of the problem are bounded, and such bounds can be approximated.
Reasons for vigilance: we can’t find (yet) any bound on the atomic (uninterruptible) optimization of the world, except the loosest bounds given by the laws of physics. And the few fields with unbounded phenomena suggest a complete phase transition in the design space when going from bounded to unbounded problems.
Direct Access: the phenomenon studied can be accessed directly through experiments.
Reasons for vigilance: Systems optimizing the world to the degree considered in alignment don’t exist yet. In addition, chilling out until we get them might not be a great idea (see the next point about iteration).
Iteration: the problem can be safely iterated upon.
Reasons for vigilance: AI risks scenarios involve massive optimization of the world in atomic ways (without us being able to interrupt). And even without leading to the end of the world, strong optimization could still bring about a catastrophe after only one try. Hence the need for guarantees before upping the optimization pressure.
Relaxed Ergodicity: the future behavior of the system, for almost all trajectories, can be well estimated by averaging over its possible behaviors now.
Reasons for vigilance: strong optimization shifts dynamics towards improbable worlds, leading to predictable errors when generalizing from the current distribution (where these worlds are negligible).
Closedness: the phenomenon can be considered by itself or within a simplified environment.
Reasons for vigilance: strong optimization would leverage side-channels, so not modeling those could hide the very problem we worry about.
Newtonian[3]: the system reacts straightforwardly to external forces applied to it (as in Newtonian mechanics), leading to predictable consequences after an intervention.
Reasons for vigilance: Systems channeling optimization, be they optimizers themselves (AGIs), composed of optimizers (markets), or under selection (cancer cells), react to interventions in convergent ways that cannot be predicted from a pure Newtonian model.
What I'm highlighting here is the need for epistemological vigilance on all these fronts. You don't have to accept the issues, just to grapple with them. If you think that one of these assumptions does hold, that's a great topic for discussion and debate. The failure mode I'm tracking is not to debate the assumptions; it's to not even consider them, while they steer us unchecked.
Thanks to Connor Leahy and TJ for discussions on these ideas. Thanks to Connor Leahy and Sid Black for feedback on a draft.
Digging into the assumptions
Boundedness: never enough
Engineers work within bounds. When you design a bridge, a software security system, a building, or a data center, what matters are the reasonable constraints on what you need to deal with: how much force, how much compute in an attack, how much temperature variation. This leads to bounds on the range of pressures and forces one has to deal with.
Such bounds ease the design process tremendously, by removing the requirement to scale forever. As an example, most cryptographic guarantees come from assuming that the attacker is only using polynomial-time computations.[4]
Yet what happens when you don’t have bounds? Alignment is in such a state right now, without bounds on the amount of optimization that the AIs will be able to do — that is, on their ability to figure things out and change the world. Physics constrains them, but with the loosest bounds possible — not much to leverage.
Unboundedness overhauls the design space. Now you have to manage every possible amount of force/pressure/optimization. Just imagine designing a security system to resist arbitrary computable attacks; none of the known cryptographic primitives we love and use would survive such a challenge.
That being said, some fields study such unbounded problems. Distributed computing theory is one, where asynchronous systems lack any bound on how long a message takes, or on the relative speed of different processes. Theoretical computer science in general tackles unboundedness in a bunch of settings (asynchronous distributed algorithms, worst-case complexity…), because modeling the exact situations in which algorithms will be used is hard, and so computer scientists aim for the strongest possible guarantees.
Epistemological vigilance for boundedness requires that we either:
find a solution that works in the unbounded setting;
find relevant and small enough bounds on capabilities and solve for this bounded setting;
or enforce such a bound on capabilities and solve for this bounded setting.
A big failure mode here is to just assume a bound that lets you prove something, when it’s not the first step to one of the three approaches above. Because we’re not trying to find versions of the problem that are easy to solve— we’re trying to solve the problem we expect to face. It’s easy to find a nice solution for a bounded setting, and simply convince oneself that the bound will hold and you will be fine. But this is not an argument, just a wish.
Direct access: so far and yet so close
If you study fluids, their physical existence helps a lot. Similarly with heat, brains, chemical substances, institutions, and computers. Your direct access to the phenomenon you’re studying lets you probe it in myriads of ways and check for yourself whether your models and theories apply. You can even amass a lot of data before making a theory.
Last time I checked, we still lacked an actual AGI, or really any way of strongly optimizing the world to the extent we worry about in alignment. So alignment research is banned from the fertile ground of interacting with the phenomenon itself. Which sucks.
It is not at all the only field of research that suffers from this problem, though: all historical sciences (evolutionary biology, geology, archaeology...) deal with it too, because their objects of study are often past events that cannot be accessed directly, witnessed, or recreated.
Most people involved in alignment acknowledge this, even when they don't agree with the rest of this list. Indeed, lack of direct access is regularly used as an argument to delay working on AGI alignment and focus instead on current systems and capabilities. That is, waiting for actual AGI or strong optimizing systems to be developed before studying them.
The problem? This proposal fails to be vigilant about the next assumption, the ability to iterate.
Iterability: don't mess it up
One thing that surprised me when reading about the Moon missions and the Apollo program is how much stuff broke all the time. The Saturn V engines pogoed, the secondary engines blew up, seams evaporated, and metal sheets warped under the (simulated) ridiculous temperature gradients of outer space. How did they manage to send people to the Moon and back alive in these conditions? Factoring out a pinch of luck, hardcore iteration. Everything was tested in as many conditions as possible, and iterated on until it didn’t break after extensive stress-tests.[5]
This incredible power of iteration can be seen in many fields where new problems need to be solved, from space engineering to drug design. When you don't know, just try out ideas and iterate. Fail faster, right?
Yet once again, alignment can’t join in on the fun. Because massive misguided optimization of the world doesn’t lend itself to a second try. If you fail, you risk game over. So epistemological vigilance tell us to either solve the problem before running the system — before iterating — or find guarantees on safety when iterating with massive amounts of optimization (which is almost the same thing as actually solving the problem).
This “you can’t get it wrong” property doesn’t crop often in science or engineering, but we can find it in the prevention of other existential risks, like nuclear war or bio-risks; or even in climate science.
The implications for alignment should be clear: we can’t just wait for the development of AGI and related technologies, and we have to work on alignment now (be it for solving the full problem or for showing that you can iterate safely), thus grappling in full with the lack of direct access.
Relaxed ergodicity: a whole new future
Imagine you’re studying gas molecules in a box. In this case and for many other systems, the dynamics behave well enough (with ergodicity for example) to let you predict relevant properties of the future states based on a deep model of the current state. Much of Boltzmann's work in statistical mechanics is based on leveraging this ability to generalize. Even without the restriction of full ergodicity, many phenomena and systems evolve in ways predictable from the current possibilities (through some sort of expectation).
Wouldn't that be nice, says epistemological vigilance. Yet strong optimization systematically shifts probability and so turns improbable world states into probable ones.[6] Thus what we observe now, with the technology available, will probably shift in non-trivial ways that need to be understood and dealt with. Ideas like instrumental convergence are qualitative predictions on this shift.
This is not a rare case. Even in statistical mechanics, you don’t always get ergodicity or the nice relaxations; and in the social sciences, this sort of shift is the standard, even if economic theory doesn’t seem good at addressing it. More generally, there’s a similarity with what Nassim Taleb calls Extremistan: settings where one outlier can matter more than everything that happened before (like many financial bets).
Quoting Taleb, those who don’t realize they’re in Extremistan get “played for suckers”. In alignment that would translate to only studying what we have access to now, with little conceptual work on what will happen after the distribution shifts, or how it will shift. And risk destruction because we refused to follow through on all our reasons for expecting a shift.
Closedness: everything is relevant
Science thrives on reductionism. By separating one phenomenon, one effect, from the rest of the world, we gain the ability to model it, understand it, and often reinsert it into the broader picture. From physics experiments to theoretical computer science’s simplifications, through managing confounding variables in social sciences studies, such isolation is key to insight after insight in science.
On the other hand, strong optimization is the perfect example of a phenomenon that cannot be boxed (pun intended). Epistemological vigilance reminds us that the core of the alignment problem lies in the impact of optimization over the larger world, and in the ability of optimization to utilize and leverage unexpected properties of the world left out of "the box abstraction". As such, knowing which details can be safely ignored is far more fraught than might be expected.
One field with this problem jumps to mind: computer security.[7] In it, a whole class of attacks —side-channel attacks — depends on implementation and other details generally left outside of formalizations, like the power consumption of the CPU.
But really, almost all sciences and engineering disciplines have examples where isolating the phenomenon ends up distorting it or even removing it. Recall from the introduction, the use of random sampling in sociology when selecting people to survey destroys any information that could have been collected about the fine structure of the network of relationships.
Examining closedness has been a focus of much of the theoretical part of conceptual alignment, from embedded agency to John's abstraction work. That being said, this epistemological vigilance is rarer in applied alignment researchers, maybe due to the prevalence of the closed system assumption in ML. As such, it's crucial to emphasize the need for vigilance here in order to avoid overconfidence in our models and experimental results.
Newtonian: complex reactions
Newton's laws of motion provide a whole ontology for thinking about how phenomena react to change: just compute the external forces, and you get a prediction of the result. Electromagnetism and Thermodynamics leverage this ontology in productive ways; so does much of structural engineering and material science, even some productivity writers.
In alignment on the other hand, the effect of interventions and change is far more involved, raising flags for epistemological vigilance. Beyond that, strong optimization doesn't just react to intervention by being pushed around; it instead channels itself through different paths towards the same convergent results. Deception in its many forms (for example deceptive alignment from the Risks paper) is but one generator of such highly non-newtonian behaviors.
This is far more common than I initially expected. Social sciences in general suffer from this problem, as a lot of their predictions, analysis and interventions alter the underlying dynamics of the social world they’re studying. Another example is cancer research, where intervening on some but not all signaling pathways might lead to adaptations towards the remaining pathways, instead of killing the cancer.
Keeping such a Newtonian assumption without a good model of what it's abstracting away leads to overconfidence on the applicability of interventions, and on our ability to direct the system. If we want to solve the problem and not delude ourselves, we need to grapple with the subtleties of reactions to interventions, if only to argue that they can be safely ignored.
Vicious synergies
As if the situation wasn’t difficult enough, note that there's a sort of vicious synergy between different assumptions. That is, the failure of one can undermine another.
Unboundedness undermines iterability, because we can’t bound how bad a missed first try would be.
As already discussed, lack of iterability undermines direct access, because it forces us to consider the problems before getting access.
Both openness and non-newtonian undermine relaxed ergodicity, as they allow more mechanisms leading to strong probability shifts.
Is it game over then?
Where does this leave us? My goal here is not to convince you that we are doomed; instead, I want to highlight which standard assumptions of science and research require epistemological vigilance if we are to solve the actual problem concerning us.
Such explicit deconfusion has at least three benefits:
(Focusing debate) Often people debate and disagree about related questions without being able to pinpoint the crux. What I hope this post give us is better shared handles to debate these questions.
(Model for newcomers) One of the hardest aspects of learning alignment is to not fall into the many epistemological traps that lay everywhere in the field. This post is far from sufficient to teach someone how to do that, but it is a first step.
(Open problems for epistemology of alignment) For my own research, I want a list of epistemic problems to guide me, that I can keep in mind while reading on the history of science and technology. That way, I can apply any new idea or trick I learn to all of them (as Feynman did for his own list of problems[8]), and see if they can be relevant for making alignment research go faster.
There is not much merit in solving a harder problem than what you need to solve. On the other hand, solving a simpler problem, when not in a path of attack to the actual problem, leads to inadequate solutions and overconfidence in their power. Let's hone our epistemological vigilance together, and ensure that we're moving in the best available direction.[9]
Appendix: Conjecture’s Take
This post came about from discussions within Conjecture to articulate why we think alignment is hard, and why we expect many standard ML approaches to fail. As such, our take is that each of these assumptions will break by default, and that we either need to solve the problem without them or enforce some version of them.
Nothing hampers Science and Engineering like unchecked assumptions.
As a concrete example of a field ridden with hidden premises, let's look at sociology. Sociologist must deal with the feedback of their object of study (people in social situations), their own social background, as well as the myriad of folk sociology notions floating in the memesphere. You might think that randomized surveys and statistics give you objective knowledge of the sociological world, but these tools also come with underlying assumptions — that the phenomenon under study must not depend on the fine structure of the social network, for example. In general, if you don’t realize this, you will then confidently misinterpret the results without considering the biases of your approach — as in asking kids to sort their play activities into three categories you defined in advance, and then seeing this as a “validation” of the classification.
How to avoid these mistakes? Epistemological vigilance, answer Pierre Bourdieu, Jean-Claude Chamboredon, and Jean-Claude Passeron in "Le métier de sociologue". They borrow the term from French philosopher of science Gaston Bachelard, to capture the attitude of always expliciting and questioning the assumptions behind notions, theories, models, experiments. So the naive sociologists err because they fail to maintain the restless epistemological vigilance that their field requires.
Alignment, like sociology, demands a perpetual questioning of unconscious assumptions. It’s because the alignment problem, and the way we know about it, goes against some of our most secure, obvious, and basic principles about knowledge and problem-solving. Thus we need a constant vigilance to keep them from sprouting again unnoticed and steering our work away from alignment.
In this post I thus make explicit these assumptions, and discuss why we have to be epistemologically vigilant about them.[1] Taken separately, none of these call to vigilance is specific to alignment — other fields fostered it first. What makes alignment unique is the combined undermining of all these assumptions together. Alignment researchers just can't avoid the epistemological struggle.
Here is my current list:[2]
Boundedness: the parameters of the problem are bounded, and such bounds can be approximated.
Reasons for vigilance: we can’t find (yet) any bound on the atomic (uninterruptible) optimization of the world, except the loosest bounds given by the laws of physics. And the few fields with unbounded phenomena suggest a complete phase transition in the design space when going from bounded to unbounded problems.
Direct Access: the phenomenon studied can be accessed directly through experiments.
Reasons for vigilance: Systems optimizing the world to the degree considered in alignment don’t exist yet. In addition, chilling out until we get them might not be a great idea (see the next point about iteration).
Iteration: the problem can be safely iterated upon.
Reasons for vigilance: AI risks scenarios involve massive optimization of the world in atomic ways (without us being able to interrupt). And even without leading to the end of the world, strong optimization could still bring about a catastrophe after only one try. Hence the need for guarantees before upping the optimization pressure.
Relaxed Ergodicity: the future behavior of the system, for almost all trajectories, can be well estimated by averaging over its possible behaviors now.
Reasons for vigilance: strong optimization shifts dynamics towards improbable worlds, leading to predictable errors when generalizing from the current distribution (where these worlds are negligible).
Closedness: the phenomenon can be considered by itself or within a simplified environment.
Reasons for vigilance: strong optimization would leverage side-channels, so not modeling those could hide the very problem we worry about.
Newtonian[3]: the system reacts straightforwardly to external forces applied to it (as in Newtonian mechanics), leading to predictable consequences after an intervention.
Reasons for vigilance: Systems channeling optimization, be they optimizers themselves (AGIs), composed of optimizers (markets), or under selection (cancer cells), react to interventions in convergent ways that cannot be predicted from a pure Newtonian model.
What I'm highlighting here is the need for epistemological vigilance on all these fronts. You don't have to accept the issues, just to grapple with them. If you think that one of these assumptions does hold, that's a great topic for discussion and debate. The failure mode I'm tracking is not to debate the assumptions; it's to not even consider them, while they steer us unchecked.
Thanks to Connor Leahy and TJ for discussions on these ideas. Thanks to Connor Leahy and Sid Black for feedback on a draft.
Digging into the assumptions
Boundedness: never enough
Engineers work within bounds. When you design a bridge, a software security system, a building, or a data center, what matters are the reasonable constraints on what you need to deal with: how much force, how much compute in an attack, how much temperature variation. This leads to bounds on the range of pressures and forces one has to deal with.
Such bounds ease the design process tremendously, by removing the requirement to scale forever. As an example, most cryptographic guarantees come from assuming that the attacker is only using polynomial-time computations.[4]
Yet what happens when you don’t have bounds? Alignment is in such a state right now, without bounds on the amount of optimization that the AIs will be able to do — that is, on their ability to figure things out and change the world. Physics constrains them, but with the loosest bounds possible — not much to leverage.
Unboundedness overhauls the design space. Now you have to manage every possible amount of force/pressure/optimization. Just imagine designing a security system to resist arbitrary computable attacks; none of the known cryptographic primitives we love and use would survive such a challenge.
That being said, some fields study such unbounded problems. Distributed computing theory is one, where asynchronous systems lack any bound on how long a message takes, or on the relative speed of different processes. Theoretical computer science in general tackles unboundedness in a bunch of settings (asynchronous distributed algorithms, worst-case complexity…), because modeling the exact situations in which algorithms will be used is hard, and so computer scientists aim for the strongest possible guarantees.
Epistemological vigilance for boundedness requires that we either:
find a solution that works in the unbounded setting;
find relevant and small enough bounds on capabilities and solve for this bounded setting;
or enforce such a bound on capabilities and solve for this bounded setting.
A big failure mode here is to just assume a bound that lets you prove something, when it’s not the first step to one of the three approaches above. Because we’re not trying to find versions of the problem that are easy to solve— we’re trying to solve the problem we expect to face. It’s easy to find a nice solution for a bounded setting, and simply convince oneself that the bound will hold and you will be fine. But this is not an argument, just a wish.
Direct access: so far and yet so close
If you study fluids, their physical existence helps a lot. Similarly with heat, brains, chemical substances, institutions, and computers. Your direct access to the phenomenon you’re studying lets you probe it in myriads of ways and check for yourself whether your models and theories apply. You can even amass a lot of data before making a theory.
Last time I checked, we still lacked an actual AGI, or really any way of strongly optimizing the world to the extent we worry about in alignment. So alignment research is banned from the fertile ground of interacting with the phenomenon itself. Which sucks.
It is not at all the only field of research that suffers from this problem, though: all historical sciences (evolutionary biology, geology, archaeology...) deal with it too, because their objects of study are often past events that cannot be accessed directly, witnessed, or recreated.
Most people involved in alignment acknowledge this, even when they don't agree with the rest of this list. Indeed, lack of direct access is regularly used as an argument to delay working on AGI alignment and focus instead on current systems and capabilities. That is, waiting for actual AGI or strong optimizing systems to be developed before studying them.
The problem? This proposal fails to be vigilant about the next assumption, the ability to iterate.
Iterability: don't mess it up
One thing that surprised me when reading about the Moon missions and the Apollo program is how much stuff broke all the time. The Saturn V engines pogoed, the secondary engines blew up, seams evaporated, and metal sheets warped under the (simulated) ridiculous temperature gradients of outer space. How did they manage to send people to the Moon and back alive in these conditions? Factoring out a pinch of luck, hardcore iteration. Everything was tested in as many conditions as possible, and iterated on until it didn’t break after extensive stress-tests.[5]
This incredible power of iteration can be seen in many fields where new problems need to be solved, from space engineering to drug design. When you don't know, just try out ideas and iterate. Fail faster, right?
Yet once again, alignment can’t join in on the fun. Because massive misguided optimization of the world doesn’t lend itself to a second try. If you fail, you risk game over. So epistemological vigilance tell us to either solve the problem before running the system — before iterating — or find guarantees on safety when iterating with massive amounts of optimization (which is almost the same thing as actually solving the problem).
This “you can’t get it wrong” property doesn’t crop often in science or engineering, but we can find it in the prevention of other existential risks, like nuclear war or bio-risks; or even in climate science.
The implications for alignment should be clear: we can’t just wait for the development of AGI and related technologies, and we have to work on alignment now (be it for solving the full problem or for showing that you can iterate safely), thus grappling in full with the lack of direct access.
Relaxed ergodicity: a whole new future
Imagine you’re studying gas molecules in a box. In this case and for many other systems, the dynamics behave well enough (with ergodicity for example) to let you predict relevant properties of the future states based on a deep model of the current state. Much of Boltzmann's work in statistical mechanics is based on leveraging this ability to generalize. Even without the restriction of full ergodicity, many phenomena and systems evolve in ways predictable from the current possibilities (through some sort of expectation).
Wouldn't that be nice, says epistemological vigilance. Yet strong optimization systematically shifts probability and so turns improbable world states into probable ones.[6] Thus what we observe now, with the technology available, will probably shift in non-trivial ways that need to be understood and dealt with. Ideas like instrumental convergence are qualitative predictions on this shift.
This is not a rare case. Even in statistical mechanics, you don’t always get ergodicity or the nice relaxations; and in the social sciences, this sort of shift is the standard, even if economic theory doesn’t seem good at addressing it. More generally, there’s a similarity with what Nassim Taleb calls Extremistan: settings where one outlier can matter more than everything that happened before (like many financial bets).
Quoting Taleb, those who don’t realize they’re in Extremistan get “played for suckers”. In alignment that would translate to only studying what we have access to now, with little conceptual work on what will happen after the distribution shifts, or how it will shift. And risk destruction because we refused to follow through on all our reasons for expecting a shift.
Closedness: everything is relevant
Science thrives on reductionism. By separating one phenomenon, one effect, from the rest of the world, we gain the ability to model it, understand it, and often reinsert it into the broader picture. From physics experiments to theoretical computer science’s simplifications, through managing confounding variables in social sciences studies, such isolation is key to insight after insight in science.
On the other hand, strong optimization is the perfect example of a phenomenon that cannot be boxed (pun intended). Epistemological vigilance reminds us that the core of the alignment problem lies in the impact of optimization over the larger world, and in the ability of optimization to utilize and leverage unexpected properties of the world left out of "the box abstraction". As such, knowing which details can be safely ignored is far more fraught than might be expected.
One field with this problem jumps to mind: computer security.[7] In it, a whole class of attacks —side-channel attacks — depends on implementation and other details generally left outside of formalizations, like the power consumption of the CPU.
But really, almost all sciences and engineering disciplines have examples where isolating the phenomenon ends up distorting it or even removing it. Recall from the introduction, the use of random sampling in sociology when selecting people to survey destroys any information that could have been collected about the fine structure of the network of relationships.
Examining closedness has been a focus of much of the theoretical part of conceptual alignment, from embedded agency to John's abstraction work. That being said, this epistemological vigilance is rarer in applied alignment researchers, maybe due to the prevalence of the closed system assumption in ML. As such, it's crucial to emphasize the need for vigilance here in order to avoid overconfidence in our models and experimental results.
Newtonian: complex reactions
Newton's laws of motion provide a whole ontology for thinking about how phenomena react to change: just compute the external forces, and you get a prediction of the result. Electromagnetism and Thermodynamics leverage this ontology in productive ways; so does much of structural engineering and material science, even some productivity writers.
In alignment on the other hand, the effect of interventions and change is far more involved, raising flags for epistemological vigilance. Beyond that, strong optimization doesn't just react to intervention by being pushed around; it instead channels itself through different paths towards the same convergent results. Deception in its many forms (for example deceptive alignment from the Risks paper) is but one generator of such highly non-newtonian behaviors.
This is far more common than I initially expected. Social sciences in general suffer from this problem, as a lot of their predictions, analysis and interventions alter the underlying dynamics of the social world they’re studying. Another example is cancer research, where intervening on some but not all signaling pathways might lead to adaptations towards the remaining pathways, instead of killing the cancer.
Keeping such a Newtonian assumption without a good model of what it's abstracting away leads to overconfidence on the applicability of interventions, and on our ability to direct the system. If we want to solve the problem and not delude ourselves, we need to grapple with the subtleties of reactions to interventions, if only to argue that they can be safely ignored.
Vicious synergies
As if the situation wasn’t difficult enough, note that there's a sort of vicious synergy between different assumptions. That is, the failure of one can undermine another.
Unboundedness undermines iterability, because we can’t bound how bad a missed first try would be.
As already discussed, lack of iterability undermines direct access, because it forces us to consider the problems before getting access.
Both openness and non-newtonian undermine relaxed ergodicity, as they allow more mechanisms leading to strong probability shifts.
Is it game over then?
Where does this leave us? My goal here is not to convince you that we are doomed; instead, I want to highlight which standard assumptions of science and research require epistemological vigilance if we are to solve the actual problem concerning us.
Such explicit deconfusion has at least three benefits:
(Focusing debate) Often people debate and disagree about related questions without being able to pinpoint the crux. What I hope this post give us is better shared handles to debate these questions.
(Model for newcomers) One of the hardest aspects of learning alignment is to not fall into the many epistemological traps that lay everywhere in the field. This post is far from sufficient to teach someone how to do that, but it is a first step.
(Open problems for epistemology of alignment) For my own research, I want a list of epistemic problems to guide me, that I can keep in mind while reading on the history of science and technology. That way, I can apply any new idea or trick I learn to all of them (as Feynman did for his own list of problems[8]), and see if they can be relevant for making alignment research go faster.
There is not much merit in solving a harder problem than what you need to solve. On the other hand, solving a simpler problem, when not in a path of attack to the actual problem, leads to inadequate solutions and overconfidence in their power. Let's hone our epistemological vigilance together, and ensure that we're moving in the best available direction.[9]
Appendix: Conjecture’s Take
This post came about from discussions within Conjecture to articulate why we think alignment is hard, and why we expect many standard ML approaches to fail. As such, our take is that each of these assumptions will break by default, and that we either need to solve the problem without them or enforce some version of them.
Nothing hampers Science and Engineering like unchecked assumptions.
As a concrete example of a field ridden with hidden premises, let's look at sociology. Sociologist must deal with the feedback of their object of study (people in social situations), their own social background, as well as the myriad of folk sociology notions floating in the memesphere. You might think that randomized surveys and statistics give you objective knowledge of the sociological world, but these tools also come with underlying assumptions — that the phenomenon under study must not depend on the fine structure of the social network, for example. In general, if you don’t realize this, you will then confidently misinterpret the results without considering the biases of your approach — as in asking kids to sort their play activities into three categories you defined in advance, and then seeing this as a “validation” of the classification.
How to avoid these mistakes? Epistemological vigilance, answer Pierre Bourdieu, Jean-Claude Chamboredon, and Jean-Claude Passeron in "Le métier de sociologue". They borrow the term from French philosopher of science Gaston Bachelard, to capture the attitude of always expliciting and questioning the assumptions behind notions, theories, models, experiments. So the naive sociologists err because they fail to maintain the restless epistemological vigilance that their field requires.
Alignment, like sociology, demands a perpetual questioning of unconscious assumptions. It’s because the alignment problem, and the way we know about it, goes against some of our most secure, obvious, and basic principles about knowledge and problem-solving. Thus we need a constant vigilance to keep them from sprouting again unnoticed and steering our work away from alignment.
In this post I thus make explicit these assumptions, and discuss why we have to be epistemologically vigilant about them.[1] Taken separately, none of these call to vigilance is specific to alignment — other fields fostered it first. What makes alignment unique is the combined undermining of all these assumptions together. Alignment researchers just can't avoid the epistemological struggle.
Here is my current list:[2]
Boundedness: the parameters of the problem are bounded, and such bounds can be approximated.
Reasons for vigilance: we can’t find (yet) any bound on the atomic (uninterruptible) optimization of the world, except the loosest bounds given by the laws of physics. And the few fields with unbounded phenomena suggest a complete phase transition in the design space when going from bounded to unbounded problems.
Direct Access: the phenomenon studied can be accessed directly through experiments.
Reasons for vigilance: Systems optimizing the world to the degree considered in alignment don’t exist yet. In addition, chilling out until we get them might not be a great idea (see the next point about iteration).
Iteration: the problem can be safely iterated upon.
Reasons for vigilance: AI risks scenarios involve massive optimization of the world in atomic ways (without us being able to interrupt). And even without leading to the end of the world, strong optimization could still bring about a catastrophe after only one try. Hence the need for guarantees before upping the optimization pressure.
Relaxed Ergodicity: the future behavior of the system, for almost all trajectories, can be well estimated by averaging over its possible behaviors now.
Reasons for vigilance: strong optimization shifts dynamics towards improbable worlds, leading to predictable errors when generalizing from the current distribution (where these worlds are negligible).
Closedness: the phenomenon can be considered by itself or within a simplified environment.
Reasons for vigilance: strong optimization would leverage side-channels, so not modeling those could hide the very problem we worry about.
Newtonian[3]: the system reacts straightforwardly to external forces applied to it (as in Newtonian mechanics), leading to predictable consequences after an intervention.
Reasons for vigilance: Systems channeling optimization, be they optimizers themselves (AGIs), composed of optimizers (markets), or under selection (cancer cells), react to interventions in convergent ways that cannot be predicted from a pure Newtonian model.
What I'm highlighting here is the need for epistemological vigilance on all these fronts. You don't have to accept the issues, just to grapple with them. If you think that one of these assumptions does hold, that's a great topic for discussion and debate. The failure mode I'm tracking is not to debate the assumptions; it's to not even consider them, while they steer us unchecked.
Thanks to Connor Leahy and TJ for discussions on these ideas. Thanks to Connor Leahy and Sid Black for feedback on a draft.
Digging into the assumptions
Boundedness: never enough
Engineers work within bounds. When you design a bridge, a software security system, a building, or a data center, what matters are the reasonable constraints on what you need to deal with: how much force, how much compute in an attack, how much temperature variation. This leads to bounds on the range of pressures and forces one has to deal with.
Such bounds ease the design process tremendously, by removing the requirement to scale forever. As an example, most cryptographic guarantees come from assuming that the attacker is only using polynomial-time computations.[4]
Yet what happens when you don’t have bounds? Alignment is in such a state right now, without bounds on the amount of optimization that the AIs will be able to do — that is, on their ability to figure things out and change the world. Physics constrains them, but with the loosest bounds possible — not much to leverage.
Unboundedness overhauls the design space. Now you have to manage every possible amount of force/pressure/optimization. Just imagine designing a security system to resist arbitrary computable attacks; none of the known cryptographic primitives we love and use would survive such a challenge.
That being said, some fields study such unbounded problems. Distributed computing theory is one, where asynchronous systems lack any bound on how long a message takes, or on the relative speed of different processes. Theoretical computer science in general tackles unboundedness in a bunch of settings (asynchronous distributed algorithms, worst-case complexity…), because modeling the exact situations in which algorithms will be used is hard, and so computer scientists aim for the strongest possible guarantees.
Epistemological vigilance for boundedness requires that we either:
find a solution that works in the unbounded setting;
find relevant and small enough bounds on capabilities and solve for this bounded setting;
or enforce such a bound on capabilities and solve for this bounded setting.
A big failure mode here is to just assume a bound that lets you prove something, when it’s not the first step to one of the three approaches above. Because we’re not trying to find versions of the problem that are easy to solve— we’re trying to solve the problem we expect to face. It’s easy to find a nice solution for a bounded setting, and simply convince oneself that the bound will hold and you will be fine. But this is not an argument, just a wish.
Direct access: so far and yet so close
If you study fluids, their physical existence helps a lot. Similarly with heat, brains, chemical substances, institutions, and computers. Your direct access to the phenomenon you’re studying lets you probe it in myriads of ways and check for yourself whether your models and theories apply. You can even amass a lot of data before making a theory.
Last time I checked, we still lacked an actual AGI, or really any way of strongly optimizing the world to the extent we worry about in alignment. So alignment research is banned from the fertile ground of interacting with the phenomenon itself. Which sucks.
It is not at all the only field of research that suffers from this problem, though: all historical sciences (evolutionary biology, geology, archaeology...) deal with it too, because their objects of study are often past events that cannot be accessed directly, witnessed, or recreated.
Most people involved in alignment acknowledge this, even when they don't agree with the rest of this list. Indeed, lack of direct access is regularly used as an argument to delay working on AGI alignment and focus instead on current systems and capabilities. That is, waiting for actual AGI or strong optimizing systems to be developed before studying them.
The problem? This proposal fails to be vigilant about the next assumption, the ability to iterate.
Iterability: don't mess it up
One thing that surprised me when reading about the Moon missions and the Apollo program is how much stuff broke all the time. The Saturn V engines pogoed, the secondary engines blew up, seams evaporated, and metal sheets warped under the (simulated) ridiculous temperature gradients of outer space. How did they manage to send people to the Moon and back alive in these conditions? Factoring out a pinch of luck, hardcore iteration. Everything was tested in as many conditions as possible, and iterated on until it didn’t break after extensive stress-tests.[5]
This incredible power of iteration can be seen in many fields where new problems need to be solved, from space engineering to drug design. When you don't know, just try out ideas and iterate. Fail faster, right?
Yet once again, alignment can’t join in on the fun. Because massive misguided optimization of the world doesn’t lend itself to a second try. If you fail, you risk game over. So epistemological vigilance tell us to either solve the problem before running the system — before iterating — or find guarantees on safety when iterating with massive amounts of optimization (which is almost the same thing as actually solving the problem).
This “you can’t get it wrong” property doesn’t crop often in science or engineering, but we can find it in the prevention of other existential risks, like nuclear war or bio-risks; or even in climate science.
The implications for alignment should be clear: we can’t just wait for the development of AGI and related technologies, and we have to work on alignment now (be it for solving the full problem or for showing that you can iterate safely), thus grappling in full with the lack of direct access.
Relaxed ergodicity: a whole new future
Imagine you’re studying gas molecules in a box. In this case and for many other systems, the dynamics behave well enough (with ergodicity for example) to let you predict relevant properties of the future states based on a deep model of the current state. Much of Boltzmann's work in statistical mechanics is based on leveraging this ability to generalize. Even without the restriction of full ergodicity, many phenomena and systems evolve in ways predictable from the current possibilities (through some sort of expectation).
Wouldn't that be nice, says epistemological vigilance. Yet strong optimization systematically shifts probability and so turns improbable world states into probable ones.[6] Thus what we observe now, with the technology available, will probably shift in non-trivial ways that need to be understood and dealt with. Ideas like instrumental convergence are qualitative predictions on this shift.
This is not a rare case. Even in statistical mechanics, you don’t always get ergodicity or the nice relaxations; and in the social sciences, this sort of shift is the standard, even if economic theory doesn’t seem good at addressing it. More generally, there’s a similarity with what Nassim Taleb calls Extremistan: settings where one outlier can matter more than everything that happened before (like many financial bets).
Quoting Taleb, those who don’t realize they’re in Extremistan get “played for suckers”. In alignment that would translate to only studying what we have access to now, with little conceptual work on what will happen after the distribution shifts, or how it will shift. And risk destruction because we refused to follow through on all our reasons for expecting a shift.
Closedness: everything is relevant
Science thrives on reductionism. By separating one phenomenon, one effect, from the rest of the world, we gain the ability to model it, understand it, and often reinsert it into the broader picture. From physics experiments to theoretical computer science’s simplifications, through managing confounding variables in social sciences studies, such isolation is key to insight after insight in science.
On the other hand, strong optimization is the perfect example of a phenomenon that cannot be boxed (pun intended). Epistemological vigilance reminds us that the core of the alignment problem lies in the impact of optimization over the larger world, and in the ability of optimization to utilize and leverage unexpected properties of the world left out of "the box abstraction". As such, knowing which details can be safely ignored is far more fraught than might be expected.
One field with this problem jumps to mind: computer security.[7] In it, a whole class of attacks —side-channel attacks — depends on implementation and other details generally left outside of formalizations, like the power consumption of the CPU.
But really, almost all sciences and engineering disciplines have examples where isolating the phenomenon ends up distorting it or even removing it. Recall from the introduction, the use of random sampling in sociology when selecting people to survey destroys any information that could have been collected about the fine structure of the network of relationships.
Examining closedness has been a focus of much of the theoretical part of conceptual alignment, from embedded agency to John's abstraction work. That being said, this epistemological vigilance is rarer in applied alignment researchers, maybe due to the prevalence of the closed system assumption in ML. As such, it's crucial to emphasize the need for vigilance here in order to avoid overconfidence in our models and experimental results.
Newtonian: complex reactions
Newton's laws of motion provide a whole ontology for thinking about how phenomena react to change: just compute the external forces, and you get a prediction of the result. Electromagnetism and Thermodynamics leverage this ontology in productive ways; so does much of structural engineering and material science, even some productivity writers.
In alignment on the other hand, the effect of interventions and change is far more involved, raising flags for epistemological vigilance. Beyond that, strong optimization doesn't just react to intervention by being pushed around; it instead channels itself through different paths towards the same convergent results. Deception in its many forms (for example deceptive alignment from the Risks paper) is but one generator of such highly non-newtonian behaviors.
This is far more common than I initially expected. Social sciences in general suffer from this problem, as a lot of their predictions, analysis and interventions alter the underlying dynamics of the social world they’re studying. Another example is cancer research, where intervening on some but not all signaling pathways might lead to adaptations towards the remaining pathways, instead of killing the cancer.
Keeping such a Newtonian assumption without a good model of what it's abstracting away leads to overconfidence on the applicability of interventions, and on our ability to direct the system. If we want to solve the problem and not delude ourselves, we need to grapple with the subtleties of reactions to interventions, if only to argue that they can be safely ignored.
Vicious synergies
As if the situation wasn’t difficult enough, note that there's a sort of vicious synergy between different assumptions. That is, the failure of one can undermine another.
Unboundedness undermines iterability, because we can’t bound how bad a missed first try would be.
As already discussed, lack of iterability undermines direct access, because it forces us to consider the problems before getting access.
Both openness and non-newtonian undermine relaxed ergodicity, as they allow more mechanisms leading to strong probability shifts.
Is it game over then?
Where does this leave us? My goal here is not to convince you that we are doomed; instead, I want to highlight which standard assumptions of science and research require epistemological vigilance if we are to solve the actual problem concerning us.
Such explicit deconfusion has at least three benefits:
(Focusing debate) Often people debate and disagree about related questions without being able to pinpoint the crux. What I hope this post give us is better shared handles to debate these questions.
(Model for newcomers) One of the hardest aspects of learning alignment is to not fall into the many epistemological traps that lay everywhere in the field. This post is far from sufficient to teach someone how to do that, but it is a first step.
(Open problems for epistemology of alignment) For my own research, I want a list of epistemic problems to guide me, that I can keep in mind while reading on the history of science and technology. That way, I can apply any new idea or trick I learn to all of them (as Feynman did for his own list of problems[8]), and see if they can be relevant for making alignment research go faster.
There is not much merit in solving a harder problem than what you need to solve. On the other hand, solving a simpler problem, when not in a path of attack to the actual problem, leads to inadequate solutions and overconfidence in their power. Let's hone our epistemological vigilance together, and ensure that we're moving in the best available direction.[9]
Appendix: Conjecture’s Take
This post came about from discussions within Conjecture to articulate why we think alignment is hard, and why we expect many standard ML approaches to fail. As such, our take is that each of these assumptions will break by default, and that we either need to solve the problem without them or enforce some version of them.
Latest Articles
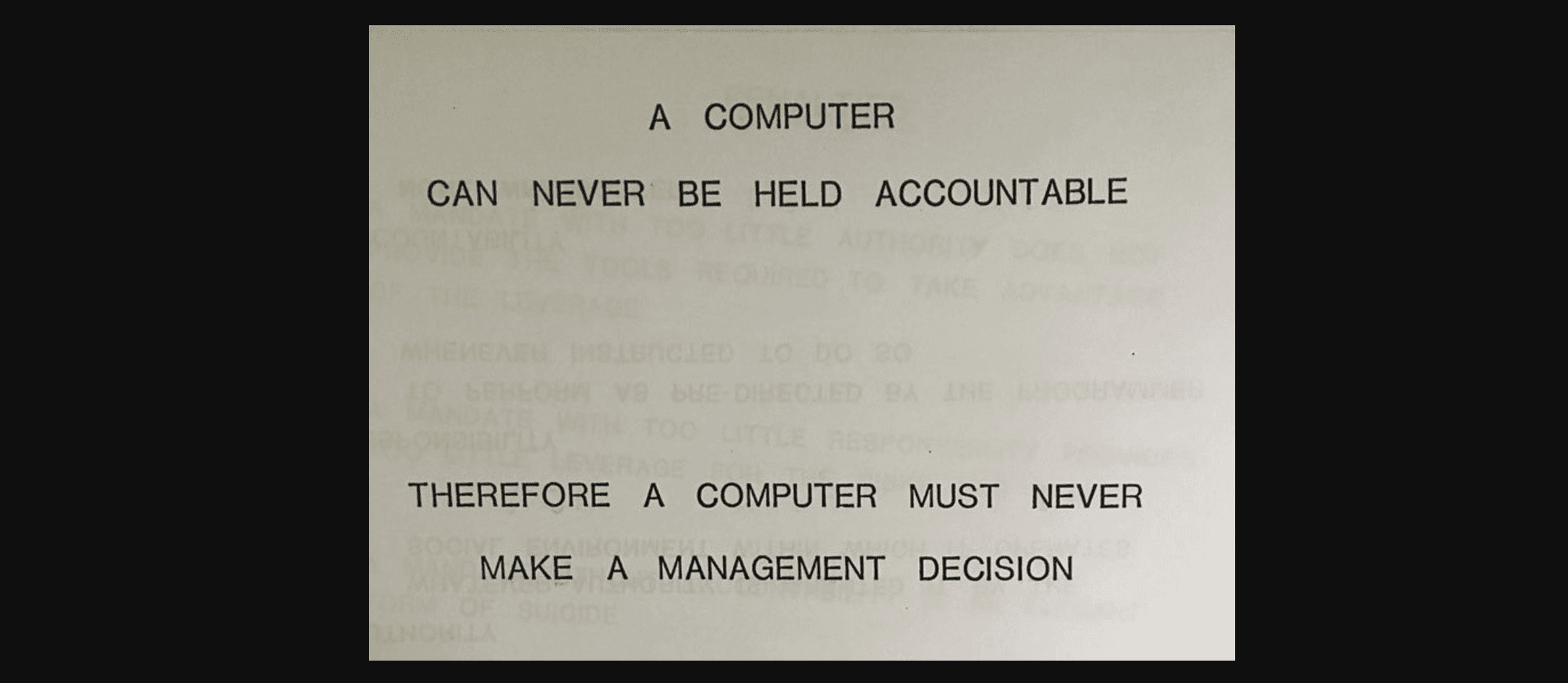
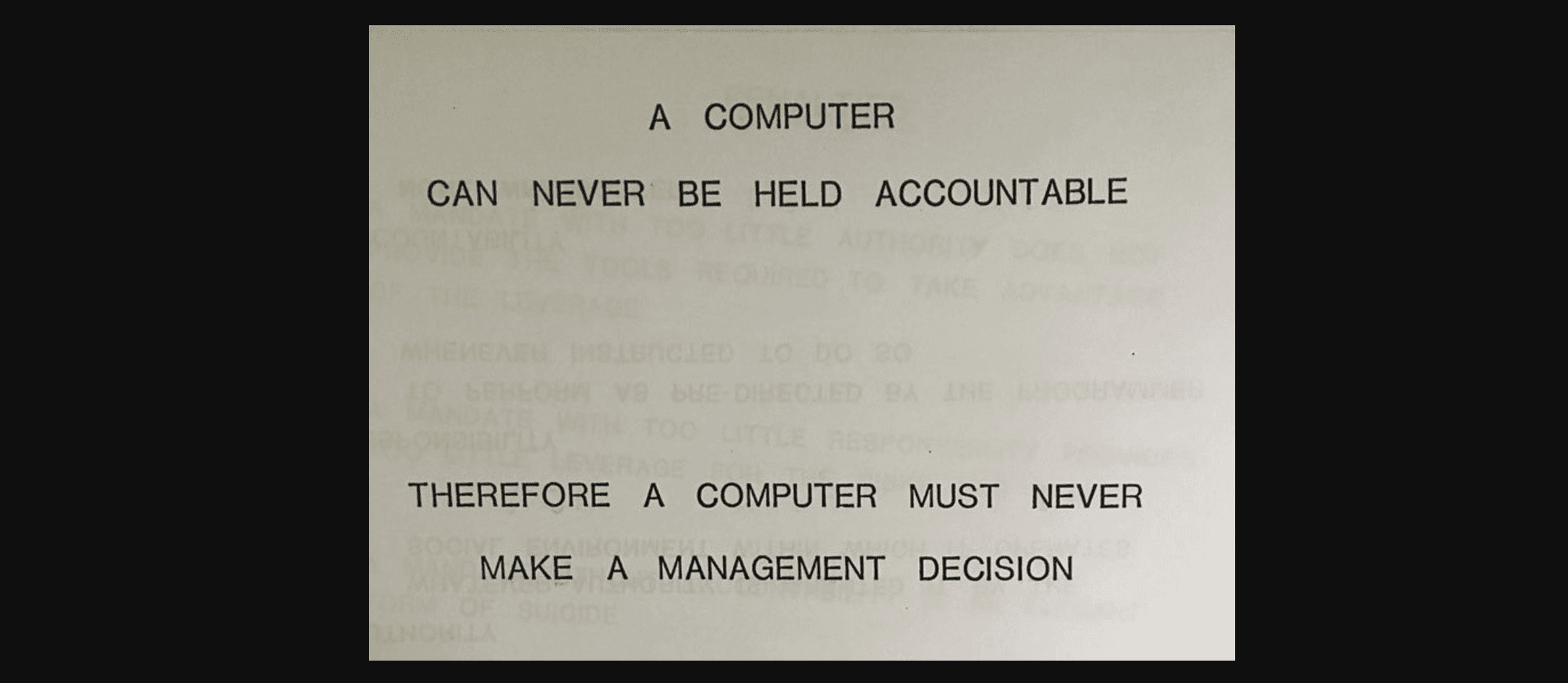
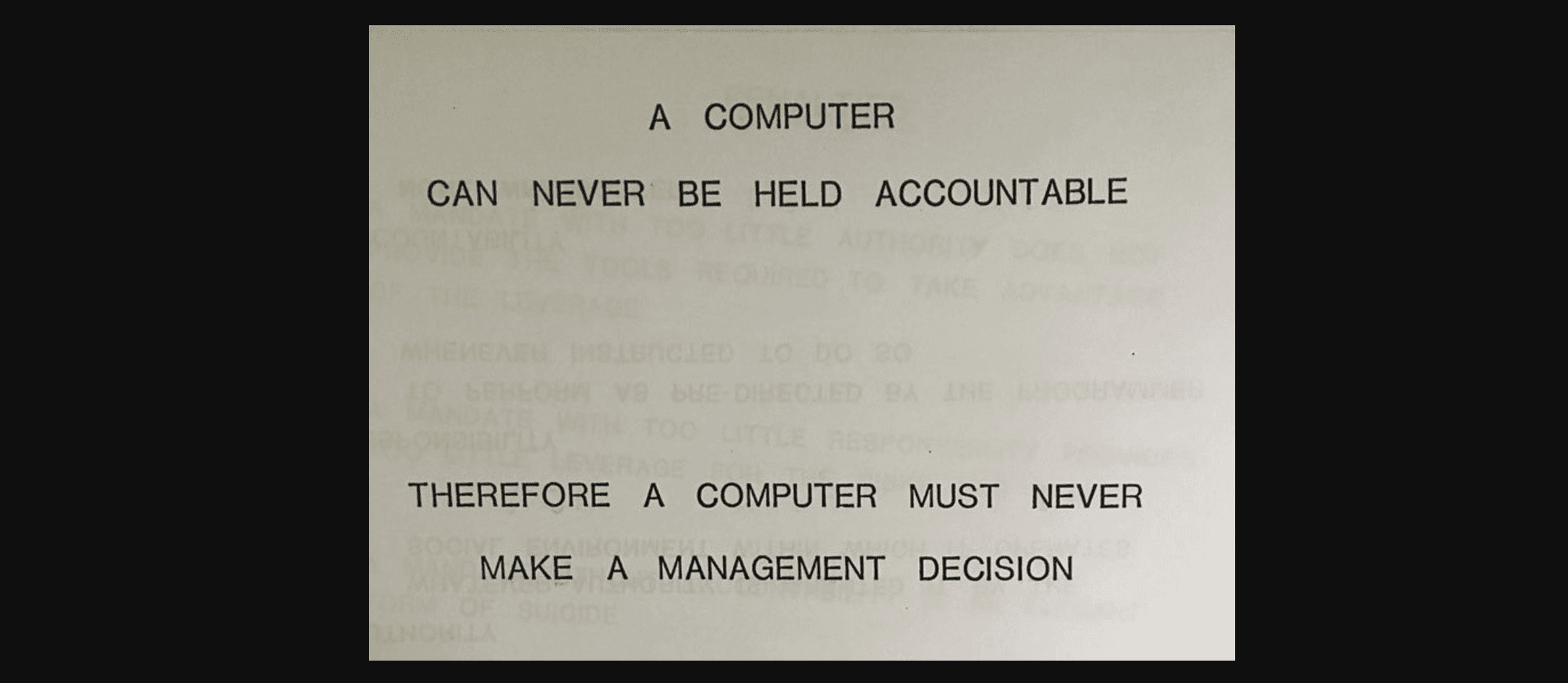
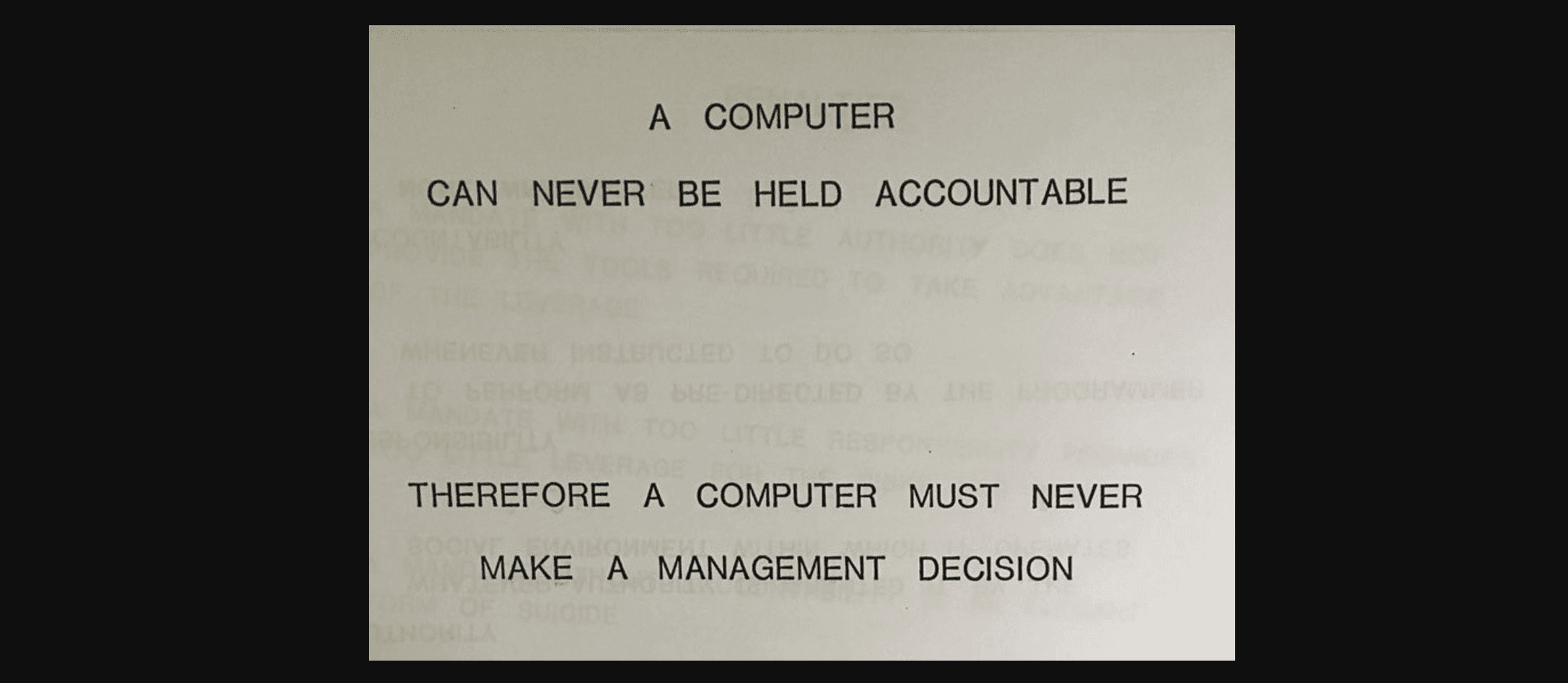
Dec 2, 2024
Conjecture: A Roadmap for Cognitive Software and A Humanist Future of AI
Conjecture: A Roadmap for Cognitive Software and A Humanist Future of AI
An overview of Conjecture's approach to "Cognitive Software," and our build path towards a good future.
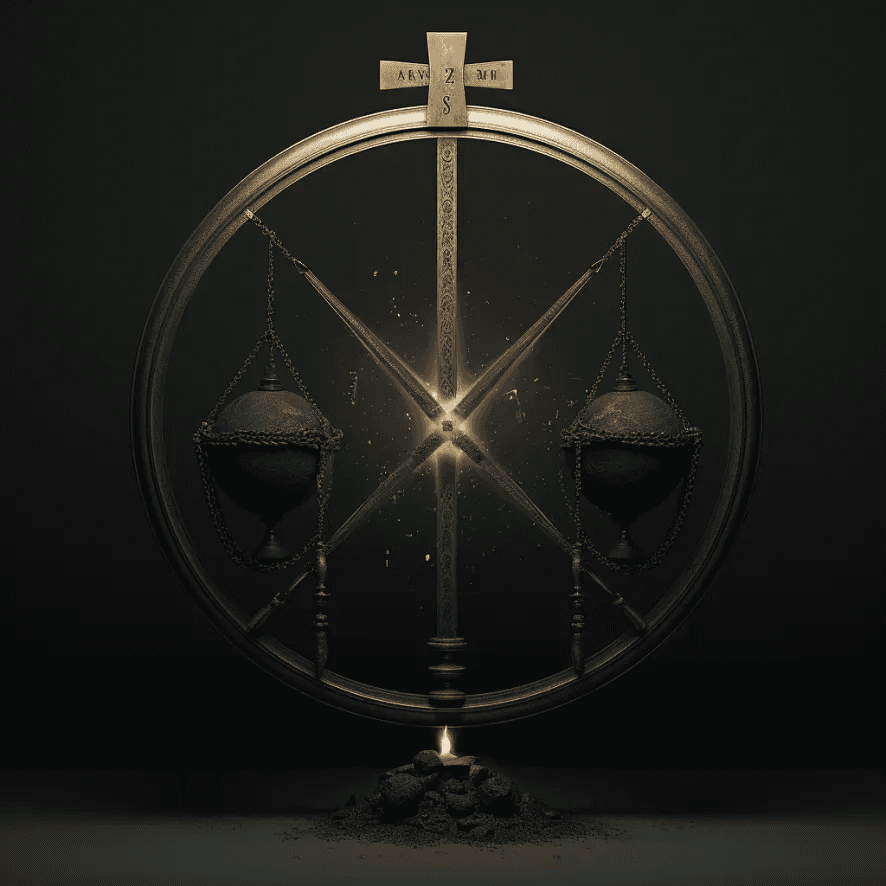
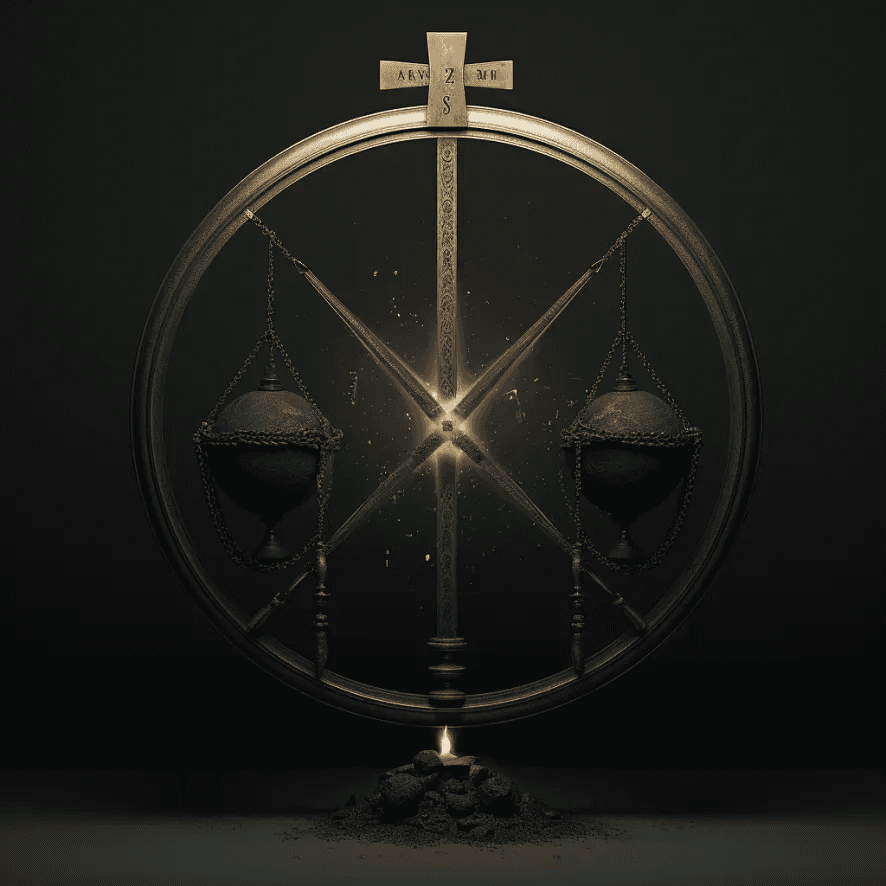
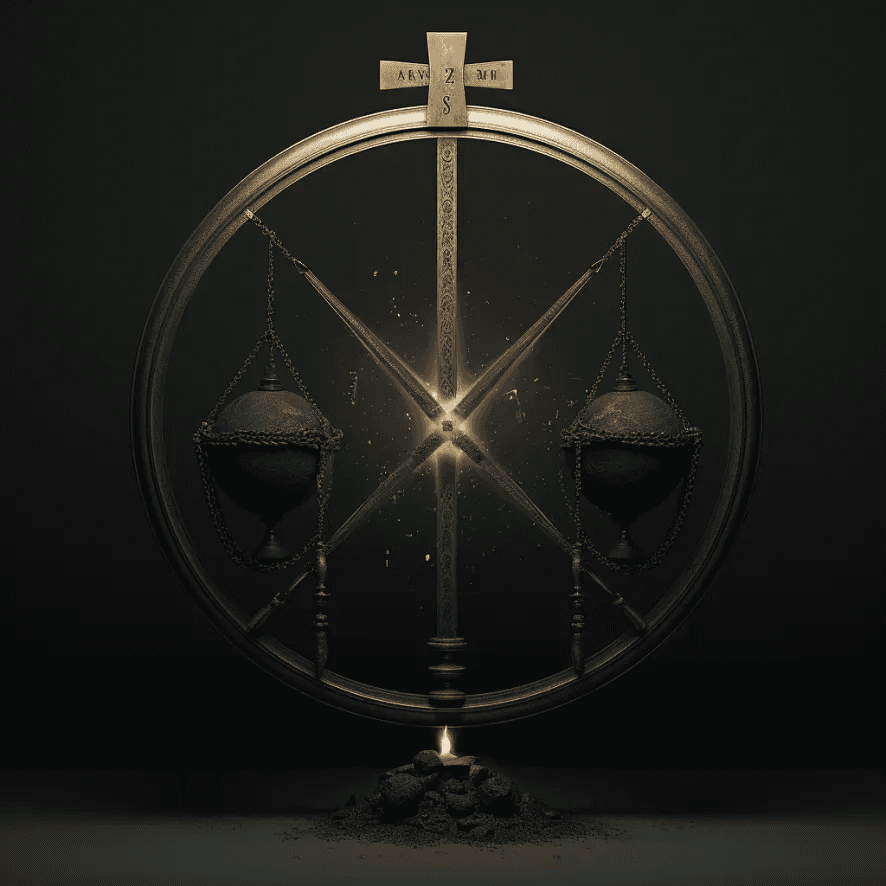
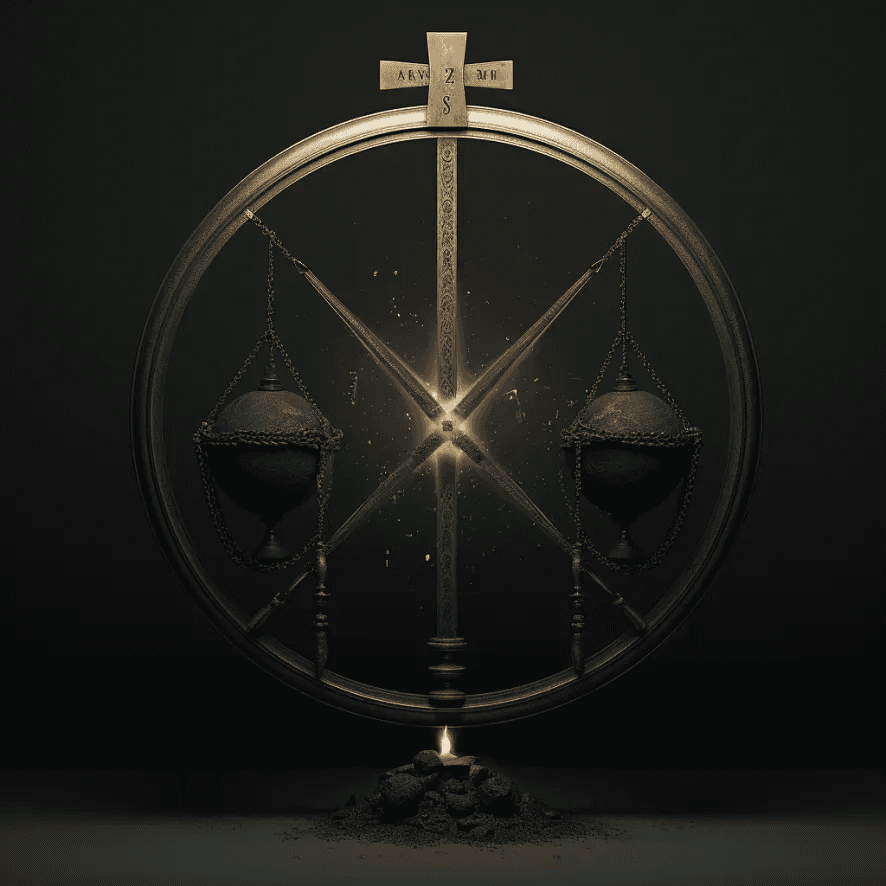
Feb 24, 2024
Christiano (ARC) and GA (Conjecture) Discuss Alignment Cruxes
Christiano (ARC) and GA (Conjecture) Discuss Alignment Cruxes
The following are the summary and transcript of a discussion between Paul Christiano (ARC) and Gabriel Alfour, hereafter GA (Conjecture), which took place on December 11, 2022 on Slack. It was held as part of a series of discussions between Conjecture and people from other organizations in the AGI and alignment field. See our retrospective on the Discussions for more information about the project and the format.
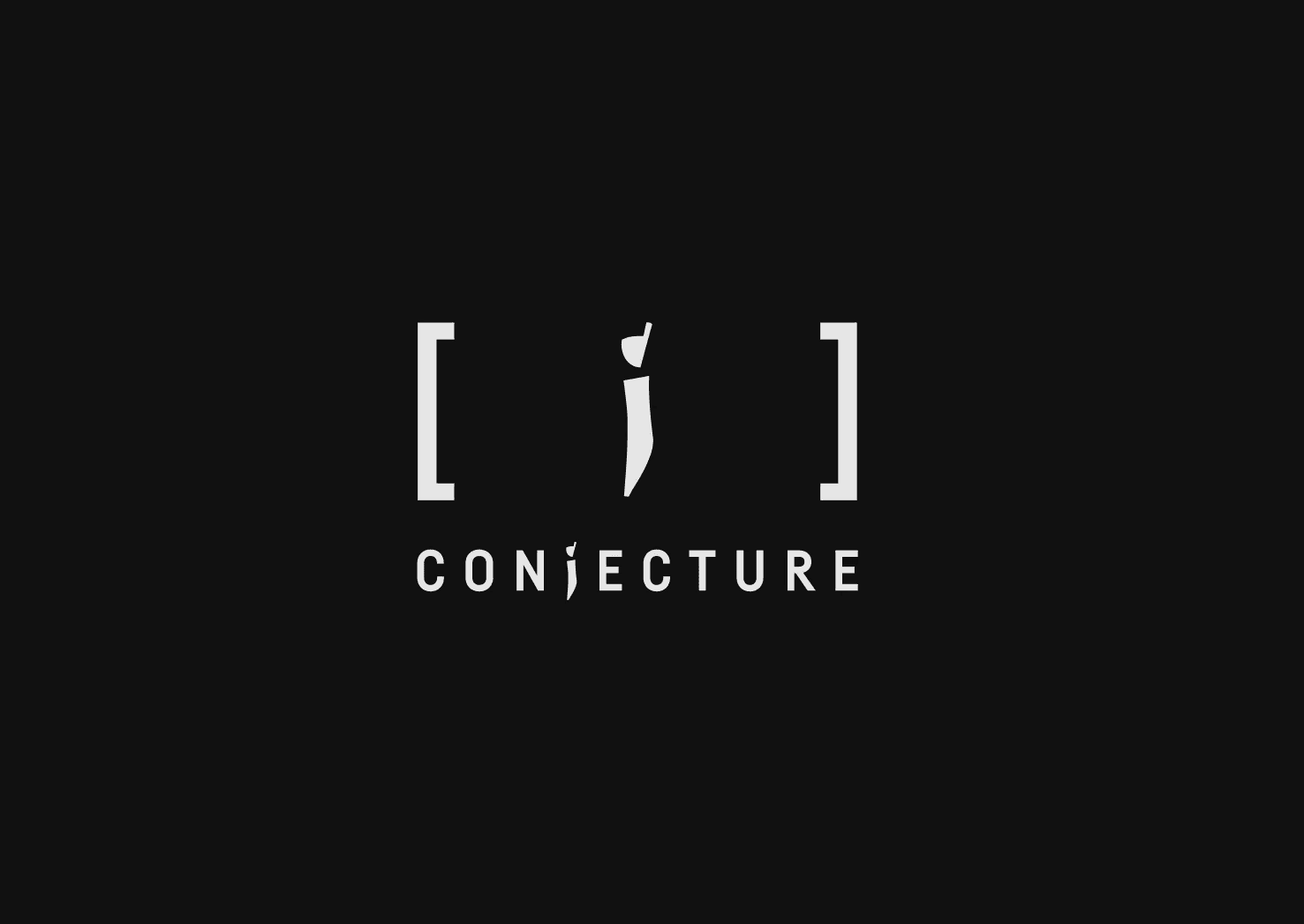
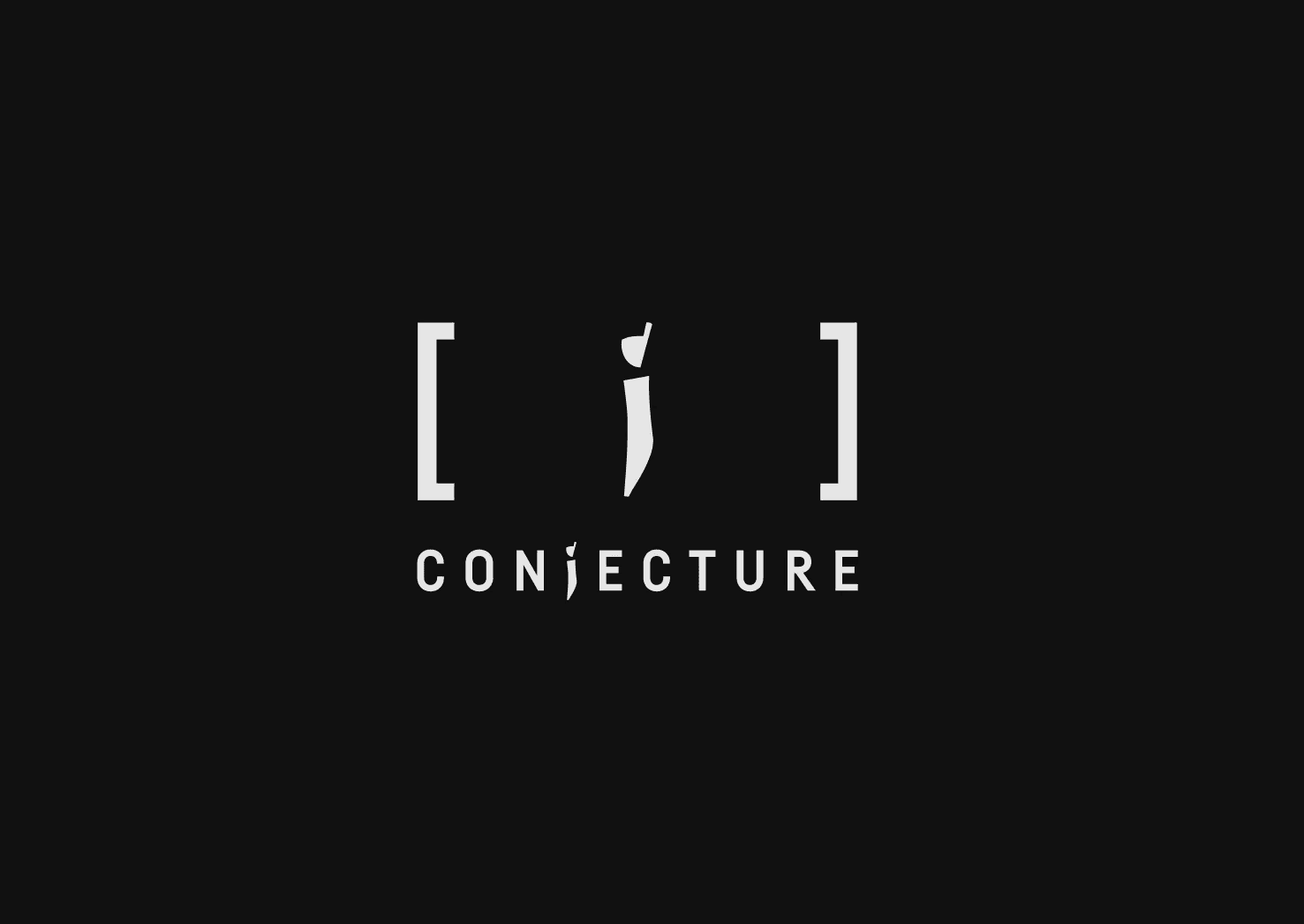
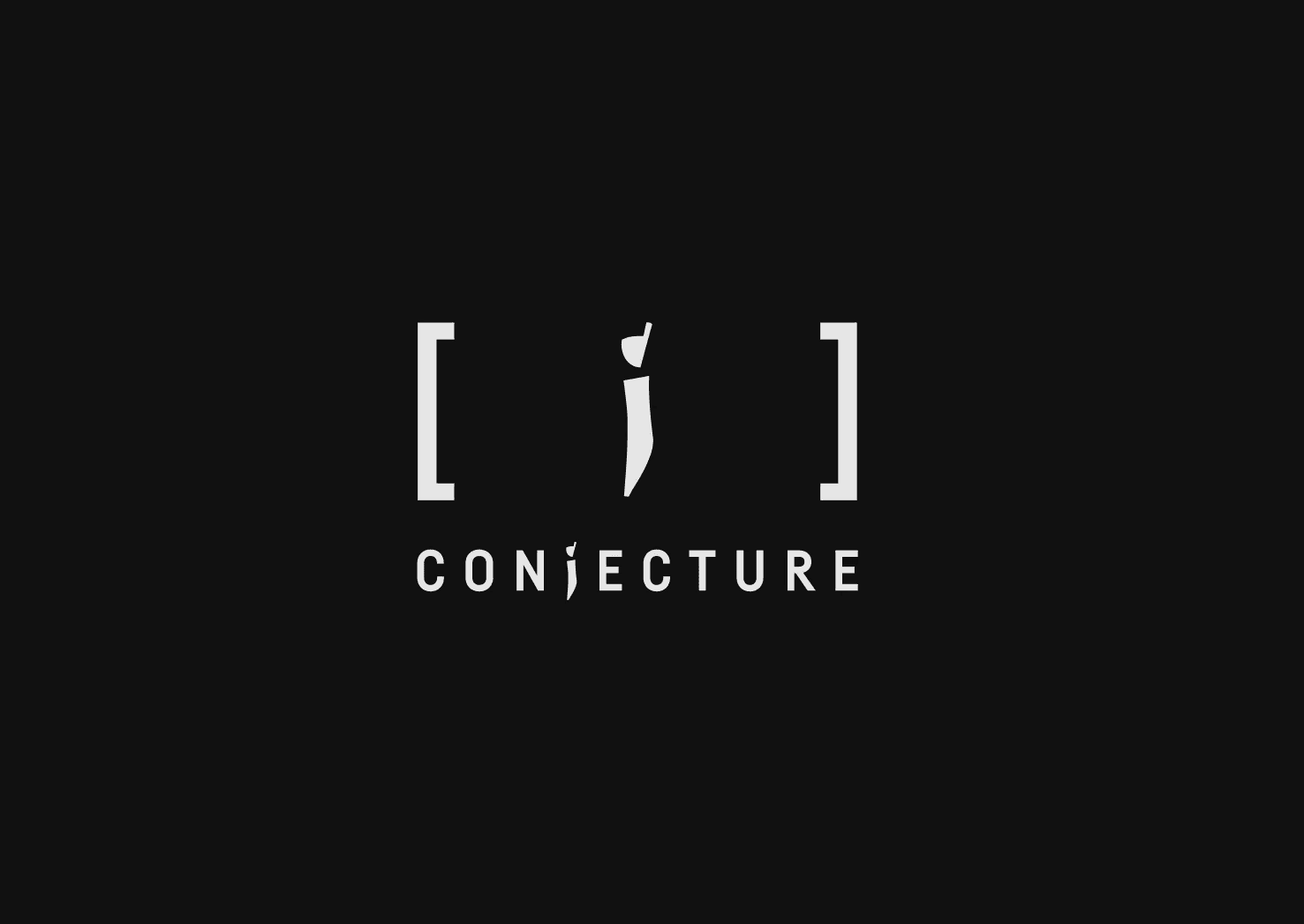
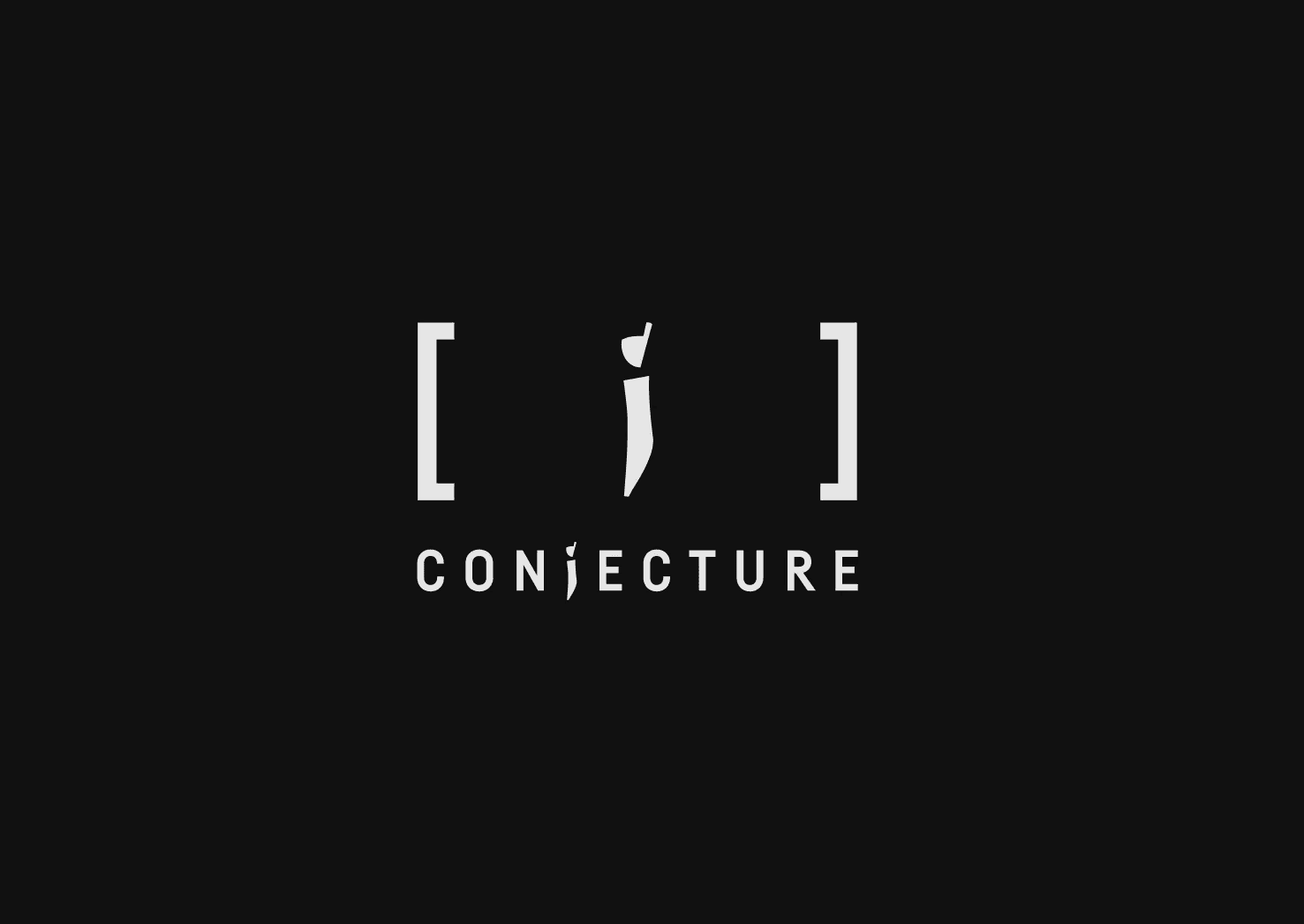
Feb 15, 2024
Conjecture: 2 Years
Conjecture: 2 Years
It has been 2 years since a group of hackers and idealists from across the globe gathered into a tiny, oxygen-deprived coworking space in downtown London with one goal in mind: Make the future go well, for everybody. And so, Conjecture was born.
Sign up to receive our newsletter and
updates on products and services.
Sign up to receive our newsletter and updates on products and services.
Sign up to receive our newsletter and updates on products and services.