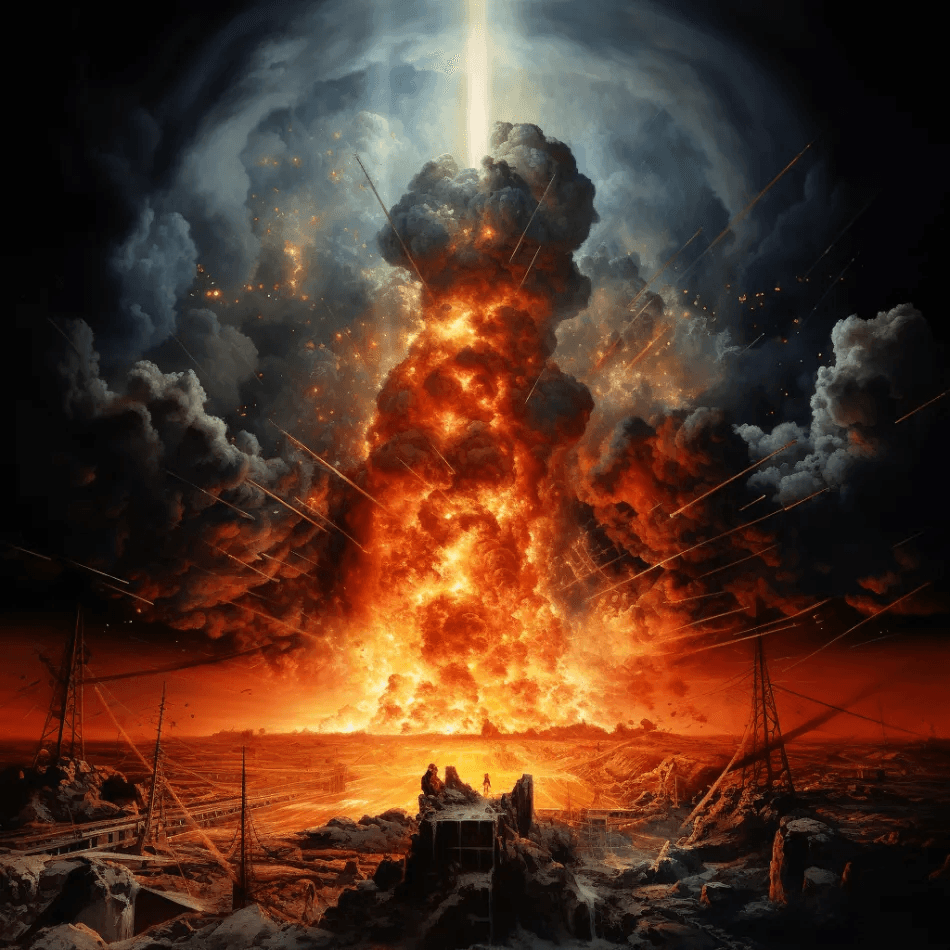
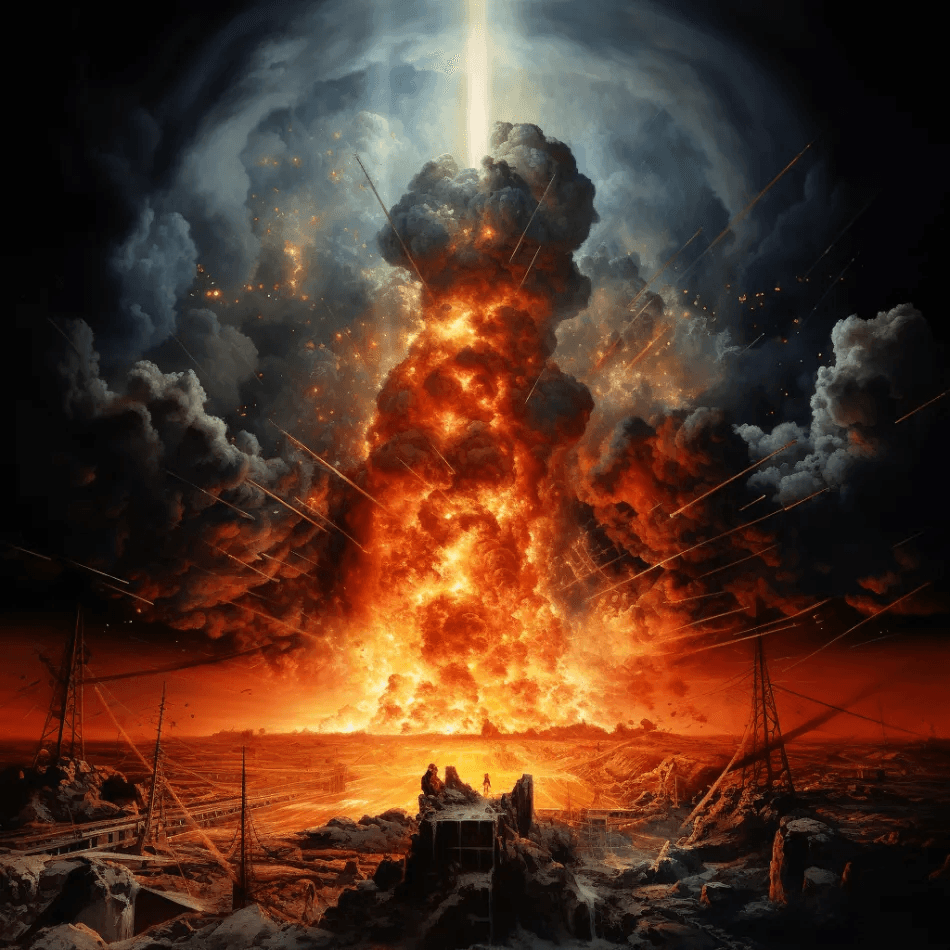
Don't accelerate problems you're trying to solve
Don't accelerate problems you're trying to solve
Don't accelerate problems you're trying to solve
Conjecture
Feb 15, 2023
If one believes that unaligned AGI is a significant problem (>10% chance of leading to catastrophe), speeding up public progress towards AGI is obviously bad.
Though it is obviously bad, there may be circumstances which require it. However, accelerating AGI should require a much higher bar of evidence and much more extreme circumstances than is commonly assumed.
There are a few categories of arguments that claim intentionally advancing AI capabilities can be helpful for alignment, which do not meet this bar[1].
Two cases of this argument are as follows
It doesn't matter much to do work that pushes capabilities if others are likely to do the same or similar work shortly after.
We should avoid capability overhangs, so that people are not surprised. To do so, we should extract as many capabilities as possible from existing AI systems.
We address these two arguments directly, arguing that the downsides are much higher than they may appear, and touch on why we believe that merely plausible arguments for advancing AI capabilities aren’t enough.
Dangerous argument 1: It doesn't matter much to do work that pushes capabilities if others are likely to do the same or similar work shortly after.
For a specific instance of this, see Paul Christiano’s “Thoughts on the impact of RLHF research”:
RLHF is just not that important to the bottom line right now. Imitation learning works nearly as well, other hacky techniques can do quite a lot to fix obvious problems […] RLHF is increasingly important as time goes on, but it also becomes increasingly overdetermined that people would have done it. In general I think your expectation should be that incidental capabilities progress from safety research is a small part of total progress […]
Markets aren’t efficient, they only approach efficiency under heavy competition when people with relevant information put effort into making them efficient. This is true for machine learning, as there aren’t that many machine learning researchers at the cutting edge, and before ChatGPT there wasn’t a ton of market pressure on them. Perhaps something as low hanging as RLHF or something similar would have happened eventually, but this isn’t generally true. Don’t assume that something seemingly obvious to you is obvious to everyone.
But even if something like RLHF or imitation learning would have happened eventually, getting small steps of progress slightly earlier can have large downstream effects. Progress often follows an s-curve, which appears exponential until the current research direction is exploited and tapers off. Moving an exponential up, even a little, early on can have large downstream consequences:
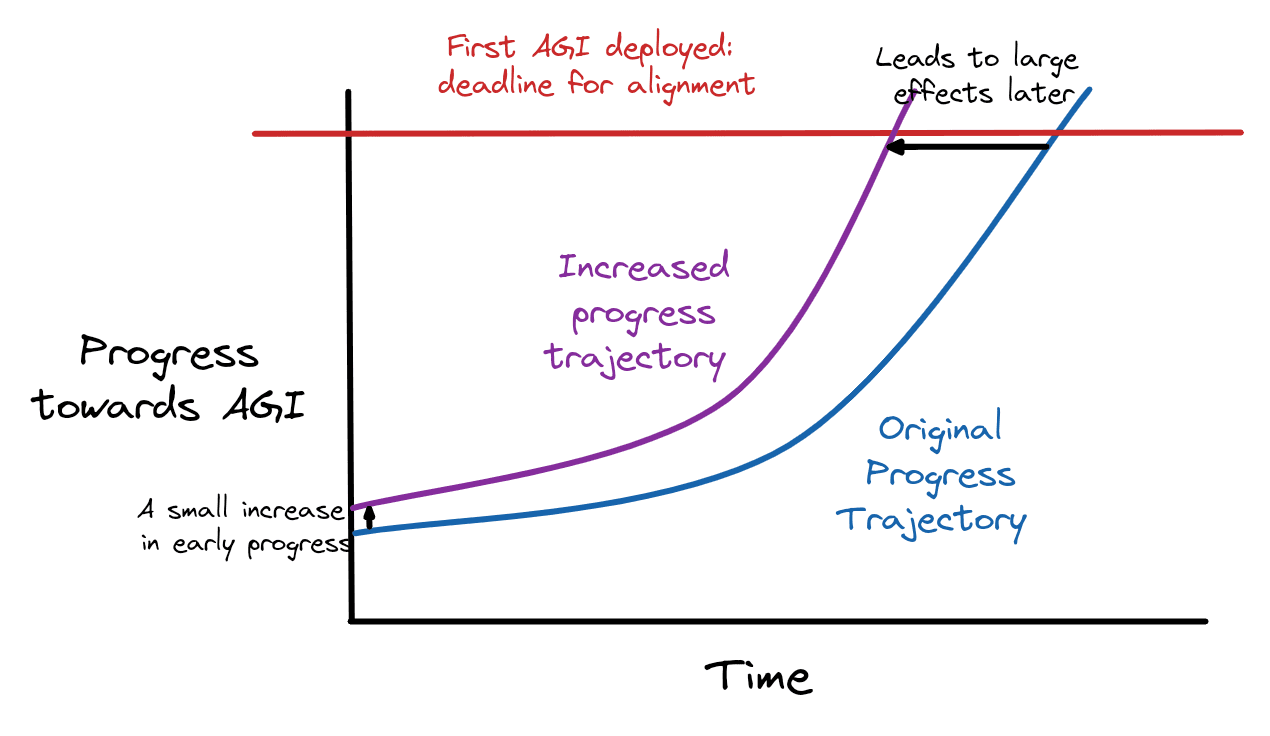
A hypothetical graph of AI capabilities progress over time. A small increase in progress early has large downstream effects due to exponential growth.
The red line indicates when the first “lethal” AGI is deployed, and thus a hard deadline for us to solve alignment. A slight increase in progress now can lead to catastrophe significantly earlier! Pushing us up the early progress exponential has really bad downstream effects!
And this is dangerous decision theory too: if every alignment researcher took a similar stance, their marginal accelerations would quickly add up.
Dangerous Argument 2: We should avoid capability overhangs, so that people are not surprised. To do so, we should extract as many capabilities as possible from existing AI systems.
Again, from Paul:
Avoiding RLHF at best introduces an important overhang: people will implicitly underestimate the capabilities of AI systems for longer, slowing progress now but leading to faster and more abrupt change later as people realize they’ve been wrong.
But there is no clear distinction between eliminating capability overhangs and discovering new capabilities. Eliminating capability overhangs is discovering AI capabilities faster, so also pushes us up the exponential! For example, it took a few years for chain-of-thought prompting to become more widely known than among a small circle of people around AI Dungeon. Once chain-of-thought became publicly known, labs started fine-tuning models to explicitly do chain-of-thought, increasing their capabilities significantly. This gap between niche discovery and public knowledge drastically slowed down progress along the growth curve!
More worryingly, we also don’t know what capabilities are safe and with what model. There exists no good theory to tell us, and a key alignment challenge is to develop such theories. Each time we advance capabilities without having solved alignment, we roll a die to see if we get catastrophic results, and each time capabilities improve, the die gets more heavily weighted against us. Perhaps GPT-5 won’t be enough to cause a catastrophe, but GPT-5 plus a bunch of other stuff might be. There is simply no way to know with our current understanding of the technology. Things that are safe on GPT-3 may not be safe on GPT-4, and if those capabilities are known earlier, future models will be built to incorporate them much earlier. Extracting all the capabilities juice from the current techniques, as soon as possible, is simply reckless.
Meta: Why arguments for advancing AI capabilities should be subject to much greater scrutiny
Advancing AI capabilities towards AGI, without a theory of alignment, is obviously bad. While all but the most extreme deontologists tend to believe that obviously bad things, such as stealing or murdering, are necessary at times, when listening to arguments for them we generally demand a much higher standard of evidence that they are necessary.
Similarly to justifying taking power or accumulating personal wealth by stealing money for yourself, finding plausible arguments for advancing AI capabilities is heavily incentivized. From VC money, to papers, prestige, hype over language model releases, beating benchmarks or solving challenges, AI capabilities are much more heavily incentivized than alignment. The weight of this money and time means that evidence for the necessity of capabilities will be found faster, and more easily.
Pushing AI capabilities for alignment is also unilateral: one group on their own can advance AI capabilities without input from other alignment groups. That makes it more likely that groups will advance AI capabilities for alignment than is ideal.
As pushing AI capabilities is both incentivized for and unilateralist, we should hold arguments for it to a much higher standard. Being merely convincing (or even worse, just plausible) isn’t enough. The case should be overwhelming.
Moreover, a lot more optimization pressure is being put into optimizing the arguments in favor of advancing capabilities than in the arguments for caution and advancing alignment, and so we should expect the epistemological terrain to be fraught in this case.
We are still engaging with the arguments as if there were not much more resources dedicated to coming up with new arguments for why pushing capabilities is good. But this is mostly for practical reasons: we expect the current community would not react well if we did not do that.
Conclusion
Advancing AI capabilities is heavily incentivized, especially when compared to alignment. As such, arguments that justify advancing capabilities as necessary for alignment should be held to a higher standard. On the other hand, there are very few alignment researchers, and they have limited resources to find and spread counter-arguments against the ones justifying capabilities. Presenting a given piece of capabilities work, as something that will happen soon enough anyways, or pushing for the elimination of “overhangs” to avoid surprise both fall under that umbrella. It is very easy to miss the downstream impacts of pushing us along the progress curve (as they are in the future), and very hard to clarify vague claims about what is an overhang versus a capability.
Instead of vague promises of helping alignment research and plausible deniability of harm, justifying advancing AI capabilities for alignment requires overwhelmingly convincing arguments that these actions will obviously advance alignment enough to be worth the downsides.
Here are a few example of arguments along these lines.
I have two major reasons to be skeptical of actively slowing down AI (setting aside feasibility):
It makes it easier for a future misaligned AI to take over by increasing overhangs, both via compute progress and algorithmic efficiency progress. (This is basically the same sort of argument as "Every 18 months, the minimum IQ necessary to destroy the world drops by one point.")
RLHF is just not that important to the bottom line right now. Imitation learning works nearly as well, other hacky techniques can do quite a lot to fix obvious problems, and the whole issue is mostly second order for the current bottom line. RLHF is increasingly important as time goes on, but it also becomes increasingly overdetermined that people would have done it. In general I think your expectation should be that incidental capabilities progress from safety research is a small part of total progress, given that it’s a small fraction of people, very much not focused on accelerating things effectively, in a domain with diminishing returns to simultaneous human effort. This can be overturned by looking at details in particular cases, but I think safety people making this argument mostly aren’t engaging with details in a realistic way
[...]
Avoiding RLHF at best introduces an important overhang: people will implicitly underestimate the capabilities of AI systems for longer, slowing progress now but leading to faster and more abrupt change later as people realize they’ve been wrong...
Paul and Rohin outlining these positions publicly is extremely laudable. We engage with Paul's post here as he makes concrete, public arguments, not to single him out.
If one believes that unaligned AGI is a significant problem (>10% chance of leading to catastrophe), speeding up public progress towards AGI is obviously bad.
Though it is obviously bad, there may be circumstances which require it. However, accelerating AGI should require a much higher bar of evidence and much more extreme circumstances than is commonly assumed.
There are a few categories of arguments that claim intentionally advancing AI capabilities can be helpful for alignment, which do not meet this bar[1].
Two cases of this argument are as follows
It doesn't matter much to do work that pushes capabilities if others are likely to do the same or similar work shortly after.
We should avoid capability overhangs, so that people are not surprised. To do so, we should extract as many capabilities as possible from existing AI systems.
We address these two arguments directly, arguing that the downsides are much higher than they may appear, and touch on why we believe that merely plausible arguments for advancing AI capabilities aren’t enough.
Dangerous argument 1: It doesn't matter much to do work that pushes capabilities if others are likely to do the same or similar work shortly after.
For a specific instance of this, see Paul Christiano’s “Thoughts on the impact of RLHF research”:
RLHF is just not that important to the bottom line right now. Imitation learning works nearly as well, other hacky techniques can do quite a lot to fix obvious problems […] RLHF is increasingly important as time goes on, but it also becomes increasingly overdetermined that people would have done it. In general I think your expectation should be that incidental capabilities progress from safety research is a small part of total progress […]
Markets aren’t efficient, they only approach efficiency under heavy competition when people with relevant information put effort into making them efficient. This is true for machine learning, as there aren’t that many machine learning researchers at the cutting edge, and before ChatGPT there wasn’t a ton of market pressure on them. Perhaps something as low hanging as RLHF or something similar would have happened eventually, but this isn’t generally true. Don’t assume that something seemingly obvious to you is obvious to everyone.
But even if something like RLHF or imitation learning would have happened eventually, getting small steps of progress slightly earlier can have large downstream effects. Progress often follows an s-curve, which appears exponential until the current research direction is exploited and tapers off. Moving an exponential up, even a little, early on can have large downstream consequences:
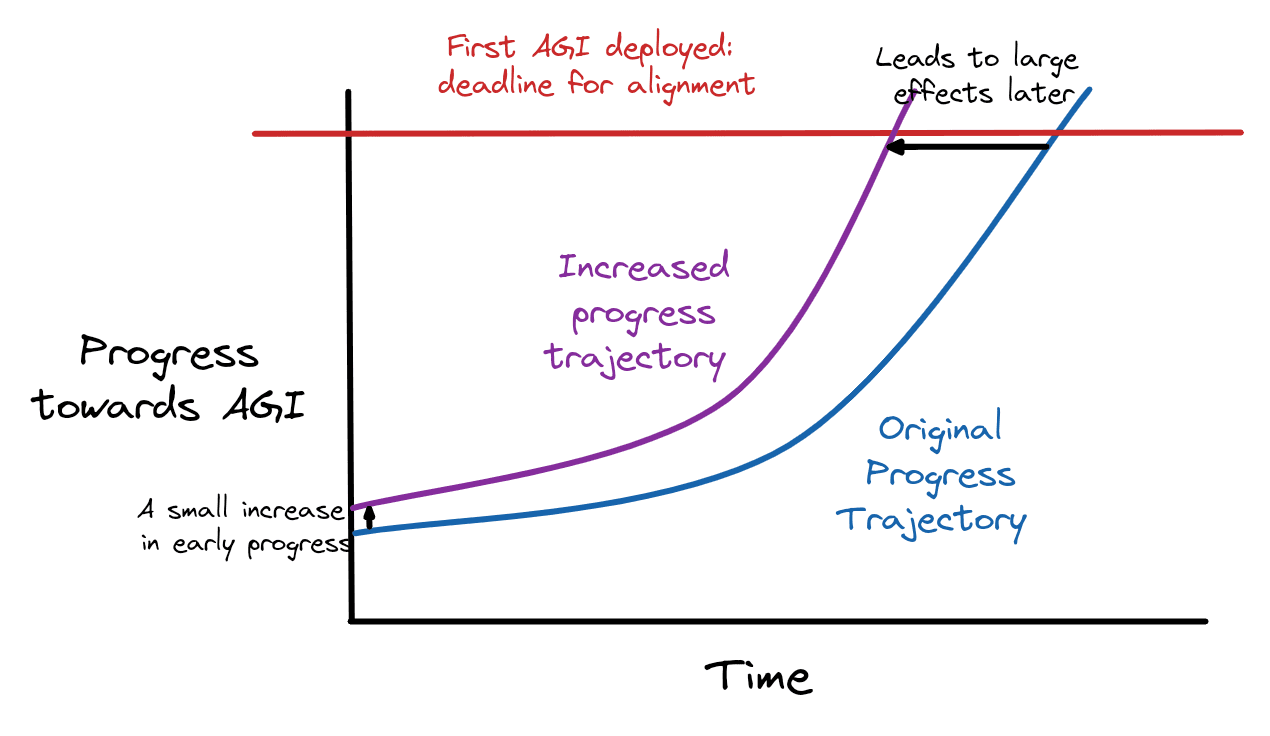
A hypothetical graph of AI capabilities progress over time. A small increase in progress early has large downstream effects due to exponential growth.
The red line indicates when the first “lethal” AGI is deployed, and thus a hard deadline for us to solve alignment. A slight increase in progress now can lead to catastrophe significantly earlier! Pushing us up the early progress exponential has really bad downstream effects!
And this is dangerous decision theory too: if every alignment researcher took a similar stance, their marginal accelerations would quickly add up.
Dangerous Argument 2: We should avoid capability overhangs, so that people are not surprised. To do so, we should extract as many capabilities as possible from existing AI systems.
Again, from Paul:
Avoiding RLHF at best introduces an important overhang: people will implicitly underestimate the capabilities of AI systems for longer, slowing progress now but leading to faster and more abrupt change later as people realize they’ve been wrong.
But there is no clear distinction between eliminating capability overhangs and discovering new capabilities. Eliminating capability overhangs is discovering AI capabilities faster, so also pushes us up the exponential! For example, it took a few years for chain-of-thought prompting to become more widely known than among a small circle of people around AI Dungeon. Once chain-of-thought became publicly known, labs started fine-tuning models to explicitly do chain-of-thought, increasing their capabilities significantly. This gap between niche discovery and public knowledge drastically slowed down progress along the growth curve!
More worryingly, we also don’t know what capabilities are safe and with what model. There exists no good theory to tell us, and a key alignment challenge is to develop such theories. Each time we advance capabilities without having solved alignment, we roll a die to see if we get catastrophic results, and each time capabilities improve, the die gets more heavily weighted against us. Perhaps GPT-5 won’t be enough to cause a catastrophe, but GPT-5 plus a bunch of other stuff might be. There is simply no way to know with our current understanding of the technology. Things that are safe on GPT-3 may not be safe on GPT-4, and if those capabilities are known earlier, future models will be built to incorporate them much earlier. Extracting all the capabilities juice from the current techniques, as soon as possible, is simply reckless.
Meta: Why arguments for advancing AI capabilities should be subject to much greater scrutiny
Advancing AI capabilities towards AGI, without a theory of alignment, is obviously bad. While all but the most extreme deontologists tend to believe that obviously bad things, such as stealing or murdering, are necessary at times, when listening to arguments for them we generally demand a much higher standard of evidence that they are necessary.
Similarly to justifying taking power or accumulating personal wealth by stealing money for yourself, finding plausible arguments for advancing AI capabilities is heavily incentivized. From VC money, to papers, prestige, hype over language model releases, beating benchmarks or solving challenges, AI capabilities are much more heavily incentivized than alignment. The weight of this money and time means that evidence for the necessity of capabilities will be found faster, and more easily.
Pushing AI capabilities for alignment is also unilateral: one group on their own can advance AI capabilities without input from other alignment groups. That makes it more likely that groups will advance AI capabilities for alignment than is ideal.
As pushing AI capabilities is both incentivized for and unilateralist, we should hold arguments for it to a much higher standard. Being merely convincing (or even worse, just plausible) isn’t enough. The case should be overwhelming.
Moreover, a lot more optimization pressure is being put into optimizing the arguments in favor of advancing capabilities than in the arguments for caution and advancing alignment, and so we should expect the epistemological terrain to be fraught in this case.
We are still engaging with the arguments as if there were not much more resources dedicated to coming up with new arguments for why pushing capabilities is good. But this is mostly for practical reasons: we expect the current community would not react well if we did not do that.
Conclusion
Advancing AI capabilities is heavily incentivized, especially when compared to alignment. As such, arguments that justify advancing capabilities as necessary for alignment should be held to a higher standard. On the other hand, there are very few alignment researchers, and they have limited resources to find and spread counter-arguments against the ones justifying capabilities. Presenting a given piece of capabilities work, as something that will happen soon enough anyways, or pushing for the elimination of “overhangs” to avoid surprise both fall under that umbrella. It is very easy to miss the downstream impacts of pushing us along the progress curve (as they are in the future), and very hard to clarify vague claims about what is an overhang versus a capability.
Instead of vague promises of helping alignment research and plausible deniability of harm, justifying advancing AI capabilities for alignment requires overwhelmingly convincing arguments that these actions will obviously advance alignment enough to be worth the downsides.
Here are a few example of arguments along these lines.
I have two major reasons to be skeptical of actively slowing down AI (setting aside feasibility):
It makes it easier for a future misaligned AI to take over by increasing overhangs, both via compute progress and algorithmic efficiency progress. (This is basically the same sort of argument as "Every 18 months, the minimum IQ necessary to destroy the world drops by one point.")
RLHF is just not that important to the bottom line right now. Imitation learning works nearly as well, other hacky techniques can do quite a lot to fix obvious problems, and the whole issue is mostly second order for the current bottom line. RLHF is increasingly important as time goes on, but it also becomes increasingly overdetermined that people would have done it. In general I think your expectation should be that incidental capabilities progress from safety research is a small part of total progress, given that it’s a small fraction of people, very much not focused on accelerating things effectively, in a domain with diminishing returns to simultaneous human effort. This can be overturned by looking at details in particular cases, but I think safety people making this argument mostly aren’t engaging with details in a realistic way
[...]
Avoiding RLHF at best introduces an important overhang: people will implicitly underestimate the capabilities of AI systems for longer, slowing progress now but leading to faster and more abrupt change later as people realize they’ve been wrong...
Paul and Rohin outlining these positions publicly is extremely laudable. We engage with Paul's post here as he makes concrete, public arguments, not to single him out.
If one believes that unaligned AGI is a significant problem (>10% chance of leading to catastrophe), speeding up public progress towards AGI is obviously bad.
Though it is obviously bad, there may be circumstances which require it. However, accelerating AGI should require a much higher bar of evidence and much more extreme circumstances than is commonly assumed.
There are a few categories of arguments that claim intentionally advancing AI capabilities can be helpful for alignment, which do not meet this bar[1].
Two cases of this argument are as follows
It doesn't matter much to do work that pushes capabilities if others are likely to do the same or similar work shortly after.
We should avoid capability overhangs, so that people are not surprised. To do so, we should extract as many capabilities as possible from existing AI systems.
We address these two arguments directly, arguing that the downsides are much higher than they may appear, and touch on why we believe that merely plausible arguments for advancing AI capabilities aren’t enough.
Dangerous argument 1: It doesn't matter much to do work that pushes capabilities if others are likely to do the same or similar work shortly after.
For a specific instance of this, see Paul Christiano’s “Thoughts on the impact of RLHF research”:
RLHF is just not that important to the bottom line right now. Imitation learning works nearly as well, other hacky techniques can do quite a lot to fix obvious problems […] RLHF is increasingly important as time goes on, but it also becomes increasingly overdetermined that people would have done it. In general I think your expectation should be that incidental capabilities progress from safety research is a small part of total progress […]
Markets aren’t efficient, they only approach efficiency under heavy competition when people with relevant information put effort into making them efficient. This is true for machine learning, as there aren’t that many machine learning researchers at the cutting edge, and before ChatGPT there wasn’t a ton of market pressure on them. Perhaps something as low hanging as RLHF or something similar would have happened eventually, but this isn’t generally true. Don’t assume that something seemingly obvious to you is obvious to everyone.
But even if something like RLHF or imitation learning would have happened eventually, getting small steps of progress slightly earlier can have large downstream effects. Progress often follows an s-curve, which appears exponential until the current research direction is exploited and tapers off. Moving an exponential up, even a little, early on can have large downstream consequences:
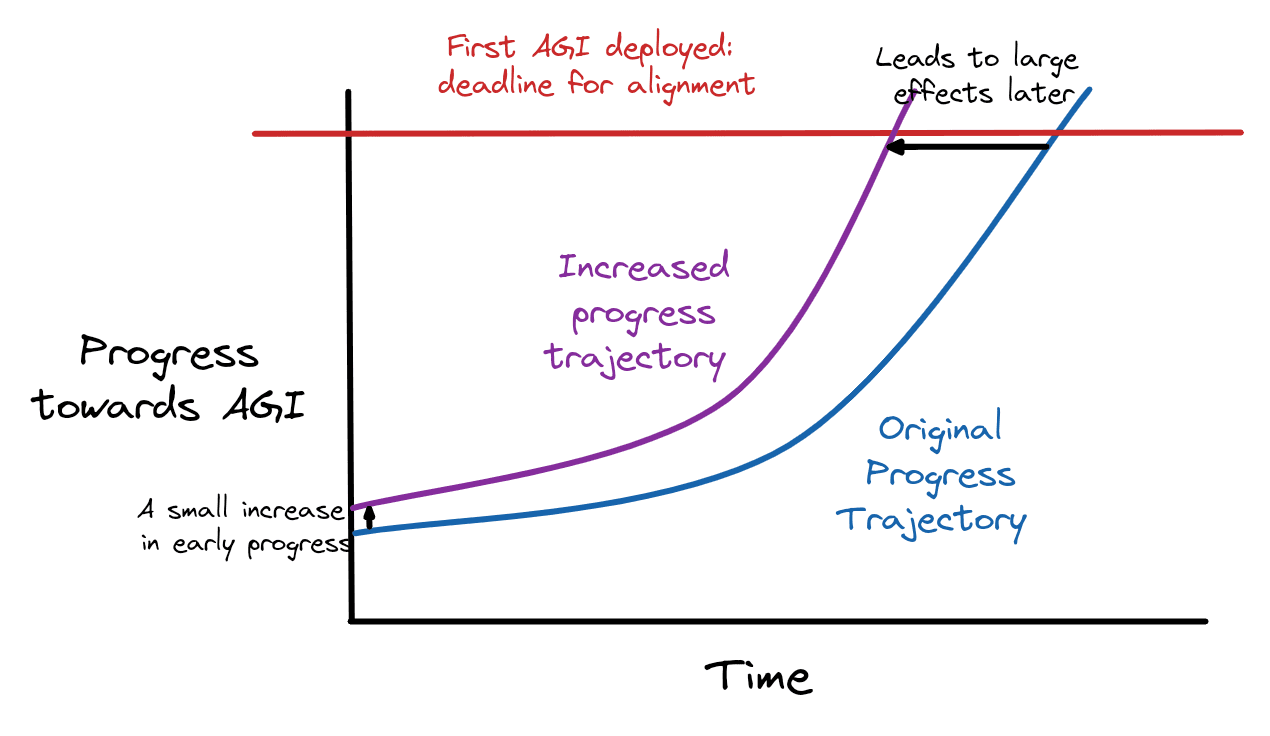
A hypothetical graph of AI capabilities progress over time. A small increase in progress early has large downstream effects due to exponential growth.
The red line indicates when the first “lethal” AGI is deployed, and thus a hard deadline for us to solve alignment. A slight increase in progress now can lead to catastrophe significantly earlier! Pushing us up the early progress exponential has really bad downstream effects!
And this is dangerous decision theory too: if every alignment researcher took a similar stance, their marginal accelerations would quickly add up.
Dangerous Argument 2: We should avoid capability overhangs, so that people are not surprised. To do so, we should extract as many capabilities as possible from existing AI systems.
Again, from Paul:
Avoiding RLHF at best introduces an important overhang: people will implicitly underestimate the capabilities of AI systems for longer, slowing progress now but leading to faster and more abrupt change later as people realize they’ve been wrong.
But there is no clear distinction between eliminating capability overhangs and discovering new capabilities. Eliminating capability overhangs is discovering AI capabilities faster, so also pushes us up the exponential! For example, it took a few years for chain-of-thought prompting to become more widely known than among a small circle of people around AI Dungeon. Once chain-of-thought became publicly known, labs started fine-tuning models to explicitly do chain-of-thought, increasing their capabilities significantly. This gap between niche discovery and public knowledge drastically slowed down progress along the growth curve!
More worryingly, we also don’t know what capabilities are safe and with what model. There exists no good theory to tell us, and a key alignment challenge is to develop such theories. Each time we advance capabilities without having solved alignment, we roll a die to see if we get catastrophic results, and each time capabilities improve, the die gets more heavily weighted against us. Perhaps GPT-5 won’t be enough to cause a catastrophe, but GPT-5 plus a bunch of other stuff might be. There is simply no way to know with our current understanding of the technology. Things that are safe on GPT-3 may not be safe on GPT-4, and if those capabilities are known earlier, future models will be built to incorporate them much earlier. Extracting all the capabilities juice from the current techniques, as soon as possible, is simply reckless.
Meta: Why arguments for advancing AI capabilities should be subject to much greater scrutiny
Advancing AI capabilities towards AGI, without a theory of alignment, is obviously bad. While all but the most extreme deontologists tend to believe that obviously bad things, such as stealing or murdering, are necessary at times, when listening to arguments for them we generally demand a much higher standard of evidence that they are necessary.
Similarly to justifying taking power or accumulating personal wealth by stealing money for yourself, finding plausible arguments for advancing AI capabilities is heavily incentivized. From VC money, to papers, prestige, hype over language model releases, beating benchmarks or solving challenges, AI capabilities are much more heavily incentivized than alignment. The weight of this money and time means that evidence for the necessity of capabilities will be found faster, and more easily.
Pushing AI capabilities for alignment is also unilateral: one group on their own can advance AI capabilities without input from other alignment groups. That makes it more likely that groups will advance AI capabilities for alignment than is ideal.
As pushing AI capabilities is both incentivized for and unilateralist, we should hold arguments for it to a much higher standard. Being merely convincing (or even worse, just plausible) isn’t enough. The case should be overwhelming.
Moreover, a lot more optimization pressure is being put into optimizing the arguments in favor of advancing capabilities than in the arguments for caution and advancing alignment, and so we should expect the epistemological terrain to be fraught in this case.
We are still engaging with the arguments as if there were not much more resources dedicated to coming up with new arguments for why pushing capabilities is good. But this is mostly for practical reasons: we expect the current community would not react well if we did not do that.
Conclusion
Advancing AI capabilities is heavily incentivized, especially when compared to alignment. As such, arguments that justify advancing capabilities as necessary for alignment should be held to a higher standard. On the other hand, there are very few alignment researchers, and they have limited resources to find and spread counter-arguments against the ones justifying capabilities. Presenting a given piece of capabilities work, as something that will happen soon enough anyways, or pushing for the elimination of “overhangs” to avoid surprise both fall under that umbrella. It is very easy to miss the downstream impacts of pushing us along the progress curve (as they are in the future), and very hard to clarify vague claims about what is an overhang versus a capability.
Instead of vague promises of helping alignment research and plausible deniability of harm, justifying advancing AI capabilities for alignment requires overwhelmingly convincing arguments that these actions will obviously advance alignment enough to be worth the downsides.
Here are a few example of arguments along these lines.
I have two major reasons to be skeptical of actively slowing down AI (setting aside feasibility):
It makes it easier for a future misaligned AI to take over by increasing overhangs, both via compute progress and algorithmic efficiency progress. (This is basically the same sort of argument as "Every 18 months, the minimum IQ necessary to destroy the world drops by one point.")
RLHF is just not that important to the bottom line right now. Imitation learning works nearly as well, other hacky techniques can do quite a lot to fix obvious problems, and the whole issue is mostly second order for the current bottom line. RLHF is increasingly important as time goes on, but it also becomes increasingly overdetermined that people would have done it. In general I think your expectation should be that incidental capabilities progress from safety research is a small part of total progress, given that it’s a small fraction of people, very much not focused on accelerating things effectively, in a domain with diminishing returns to simultaneous human effort. This can be overturned by looking at details in particular cases, but I think safety people making this argument mostly aren’t engaging with details in a realistic way
[...]
Avoiding RLHF at best introduces an important overhang: people will implicitly underestimate the capabilities of AI systems for longer, slowing progress now but leading to faster and more abrupt change later as people realize they’ve been wrong...
Paul and Rohin outlining these positions publicly is extremely laudable. We engage with Paul's post here as he makes concrete, public arguments, not to single him out.
Latest Articles
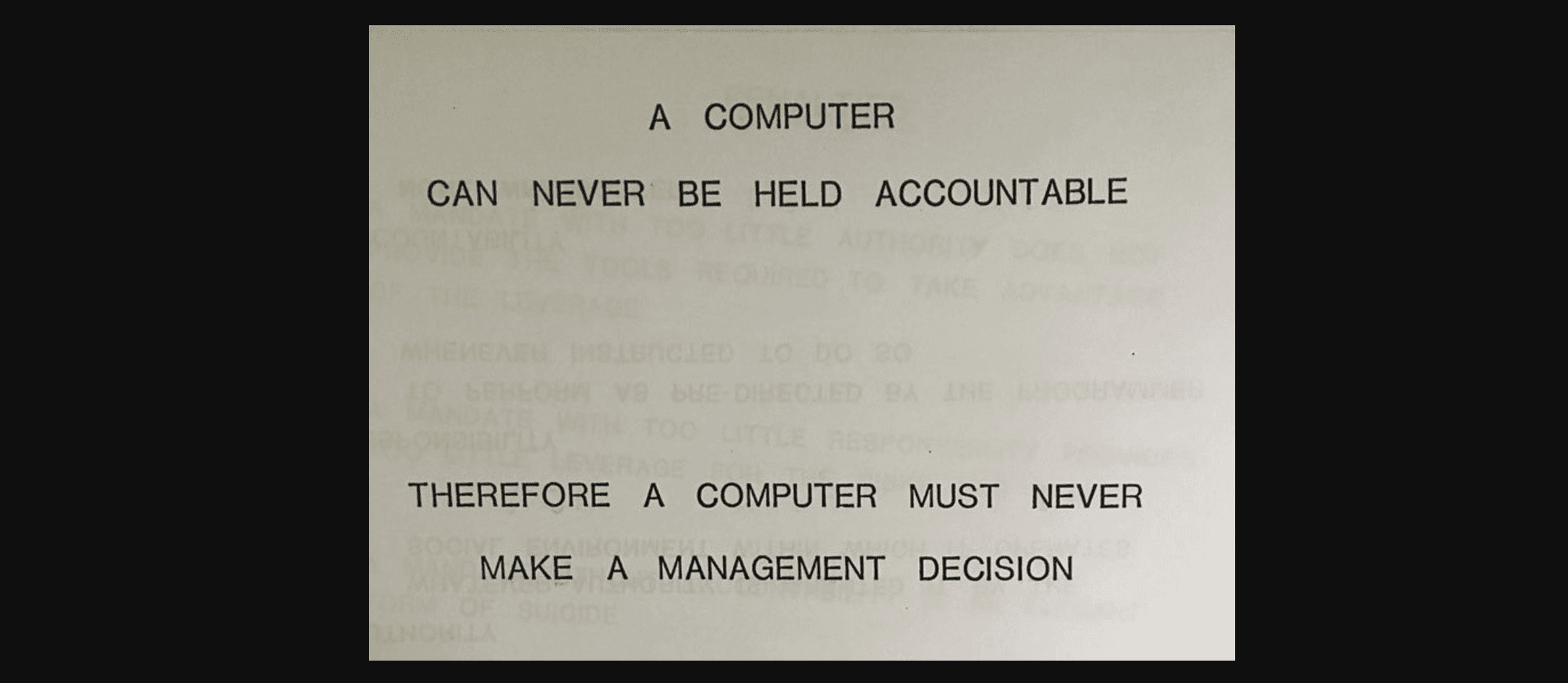
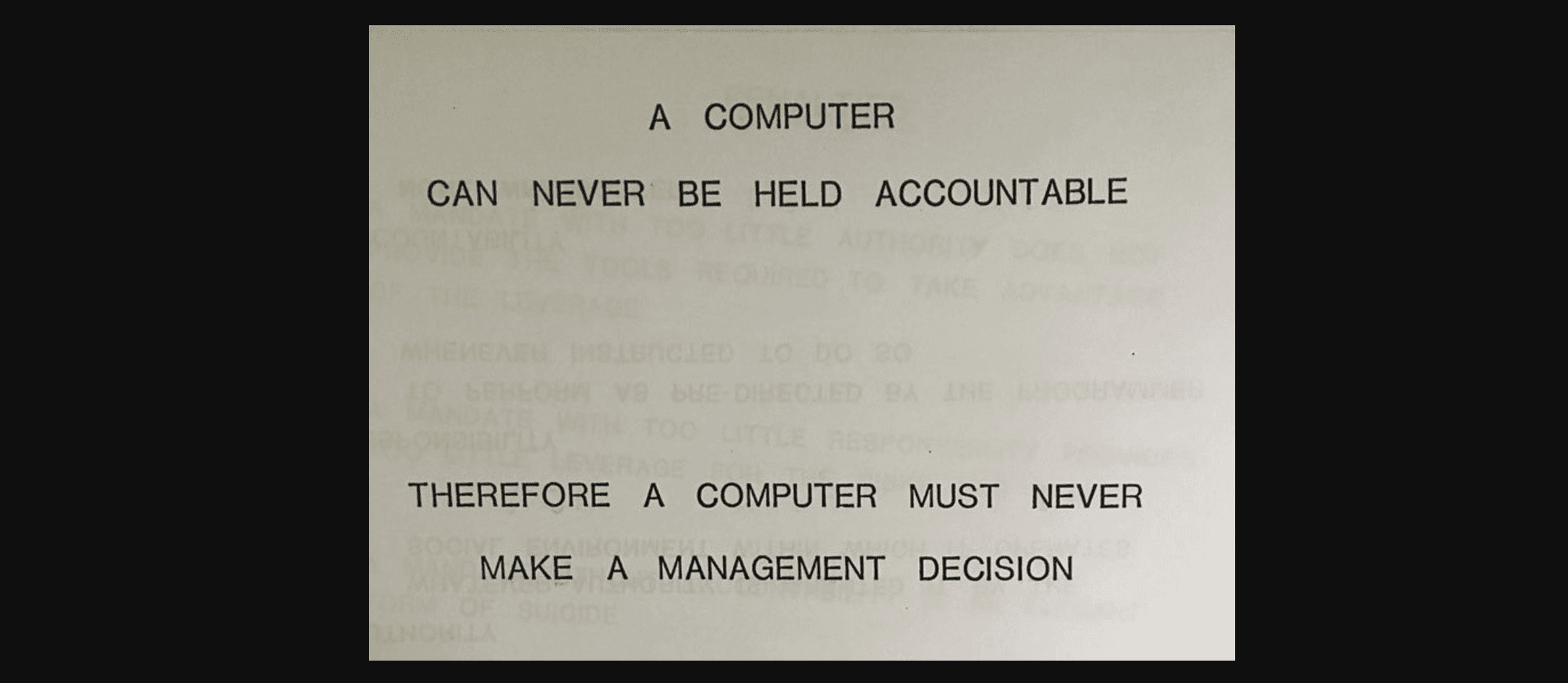
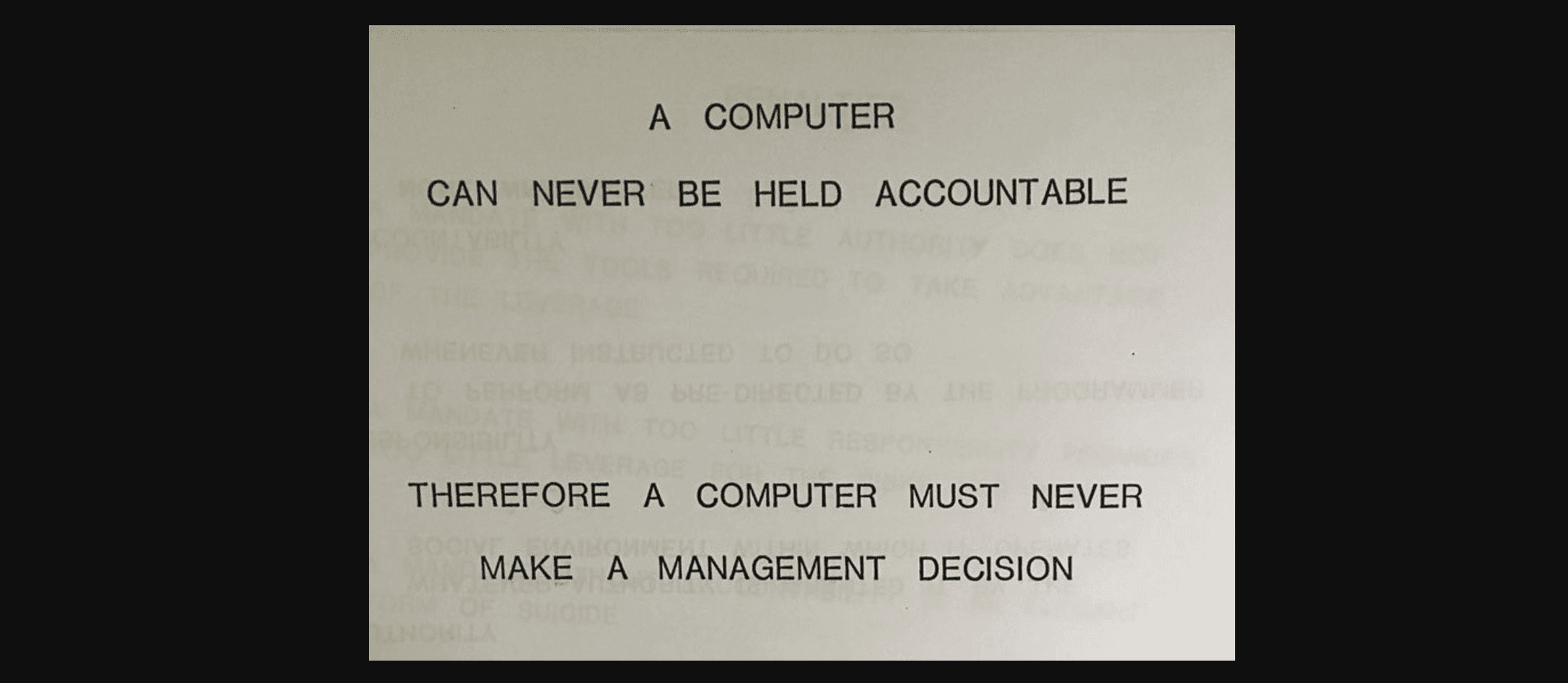
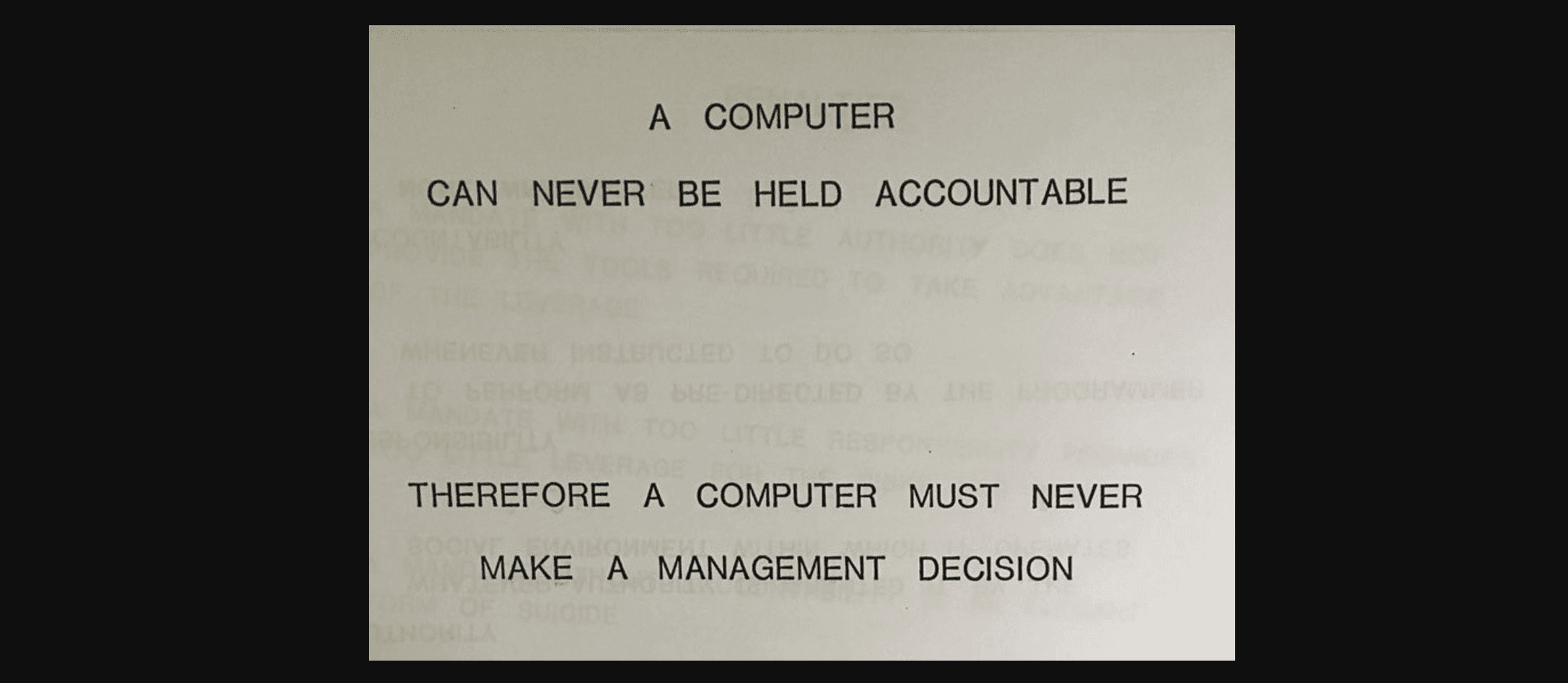
Dec 2, 2024
Conjecture: A Roadmap for Cognitive Software and A Humanist Future of AI
Conjecture: A Roadmap for Cognitive Software and A Humanist Future of AI
An overview of Conjecture's approach to "Cognitive Software," and our build path towards a good future.
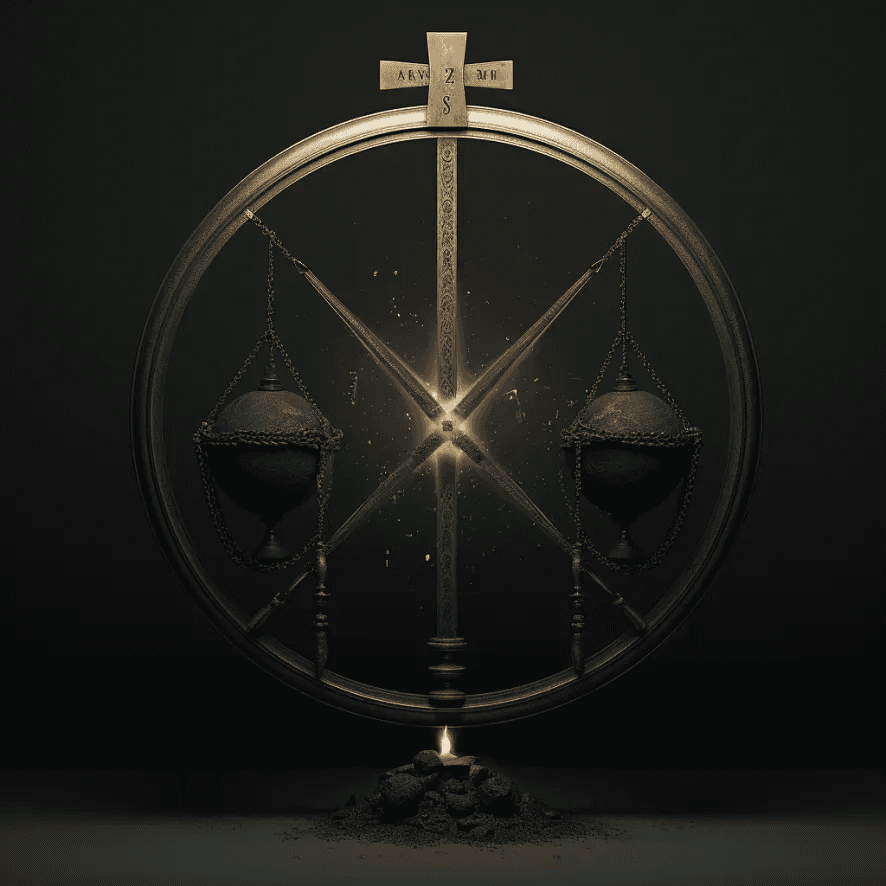
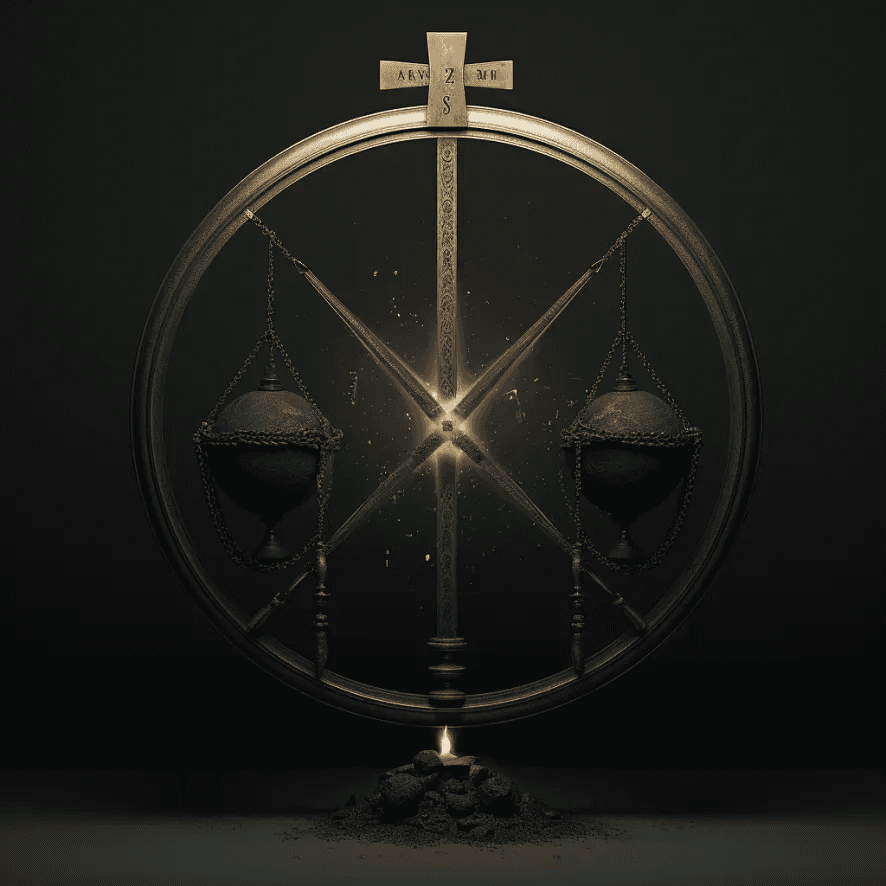
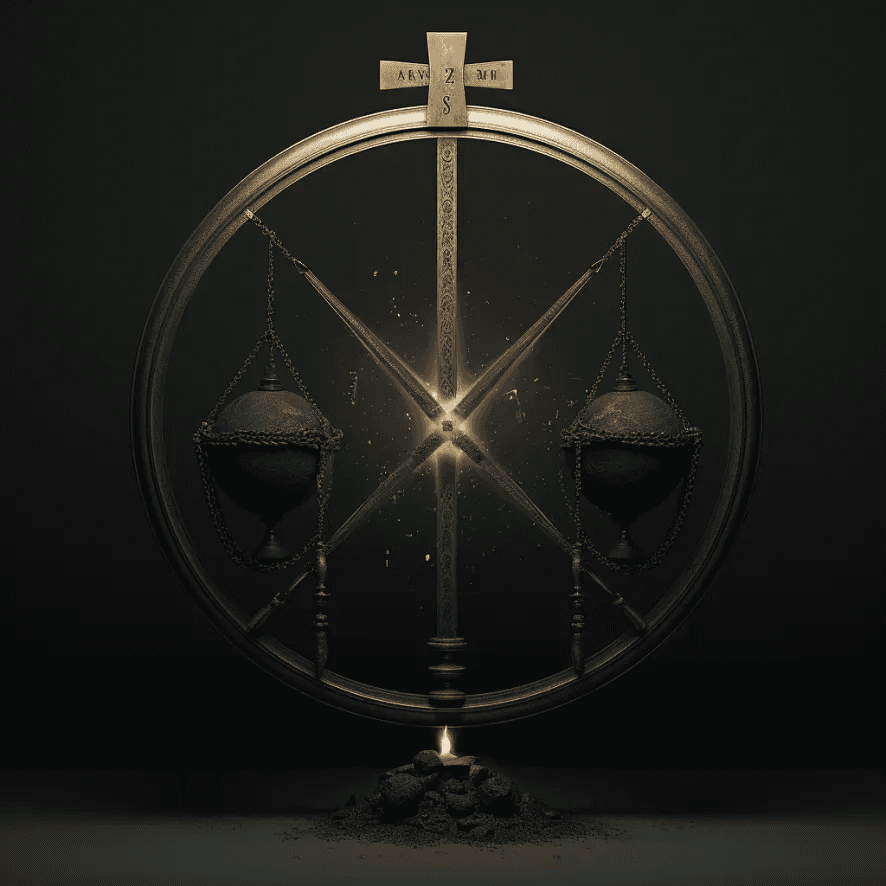
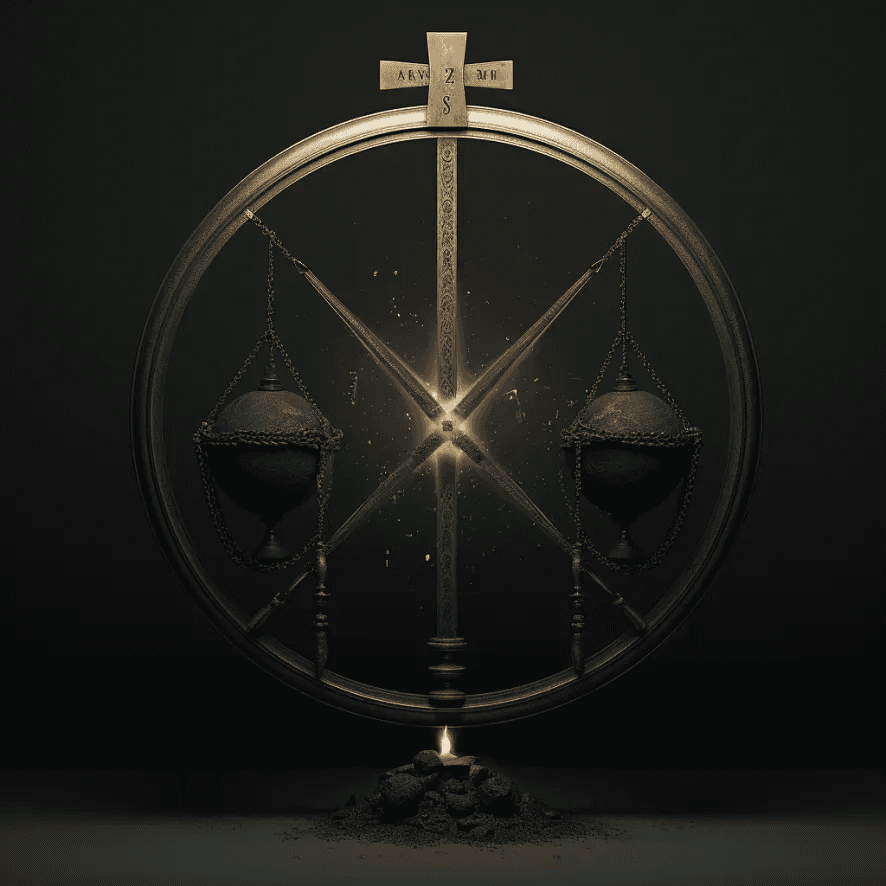
Feb 24, 2024
Christiano (ARC) and GA (Conjecture) Discuss Alignment Cruxes
Christiano (ARC) and GA (Conjecture) Discuss Alignment Cruxes
The following are the summary and transcript of a discussion between Paul Christiano (ARC) and Gabriel Alfour, hereafter GA (Conjecture), which took place on December 11, 2022 on Slack. It was held as part of a series of discussions between Conjecture and people from other organizations in the AGI and alignment field. See our retrospective on the Discussions for more information about the project and the format.
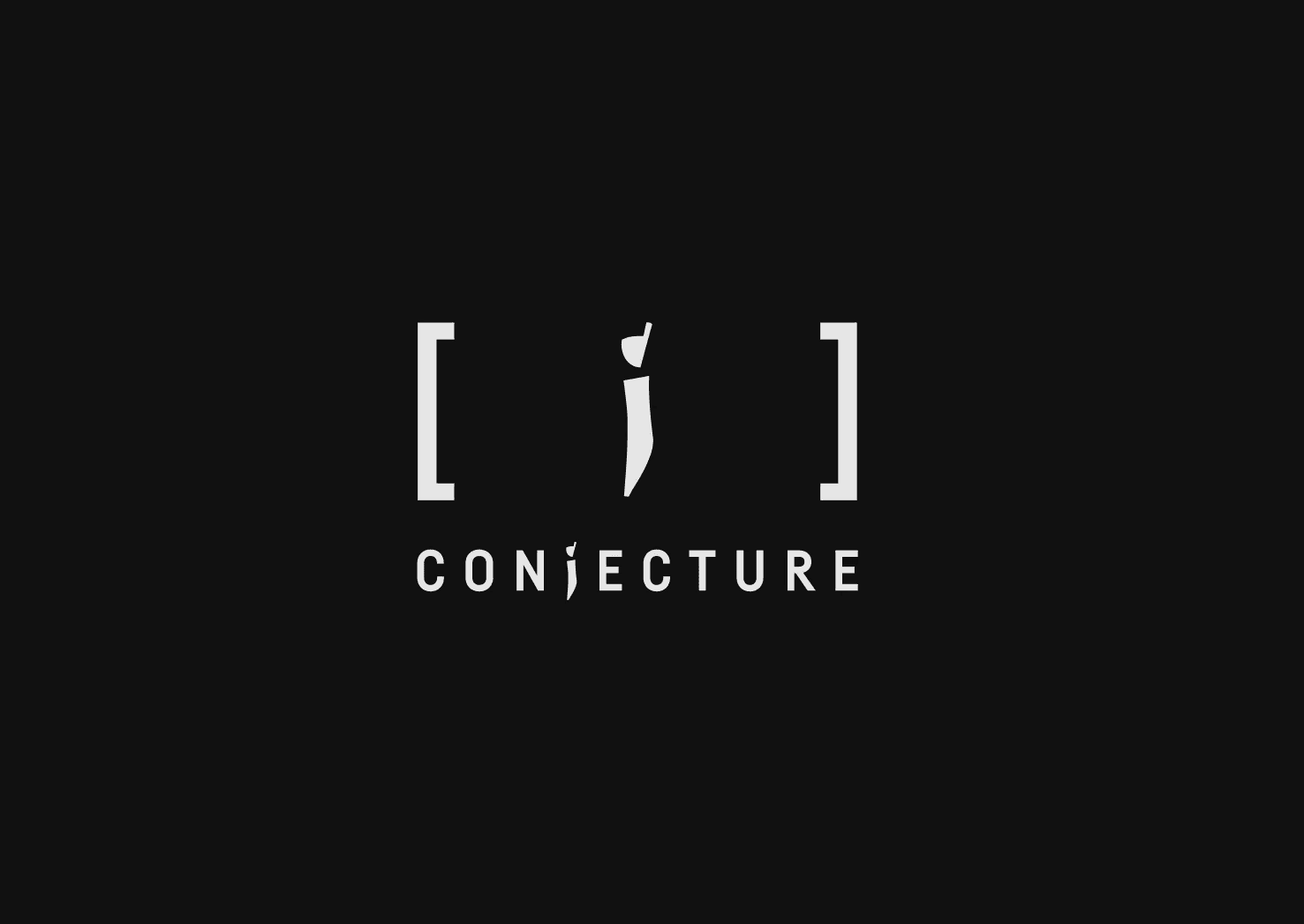
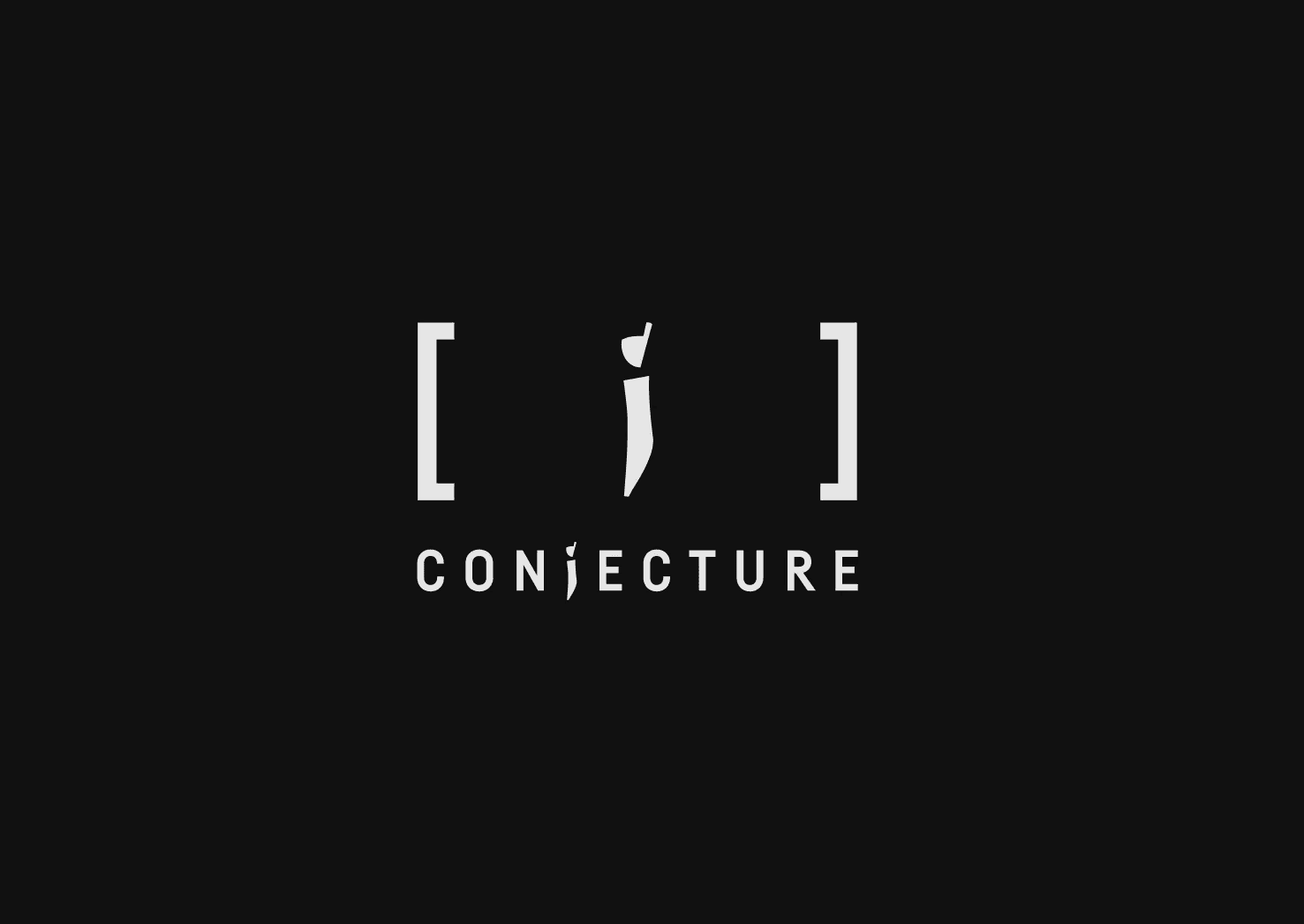
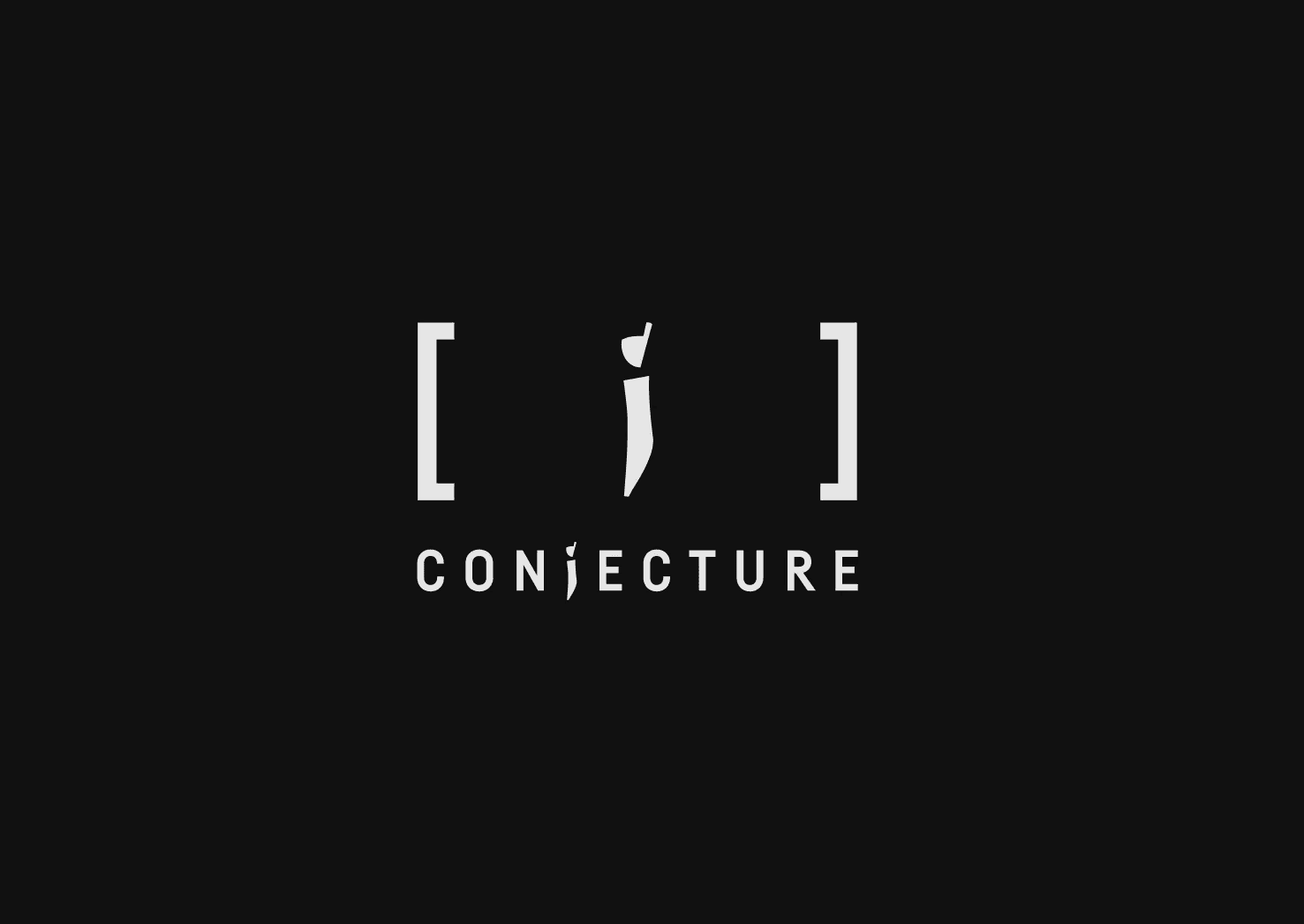
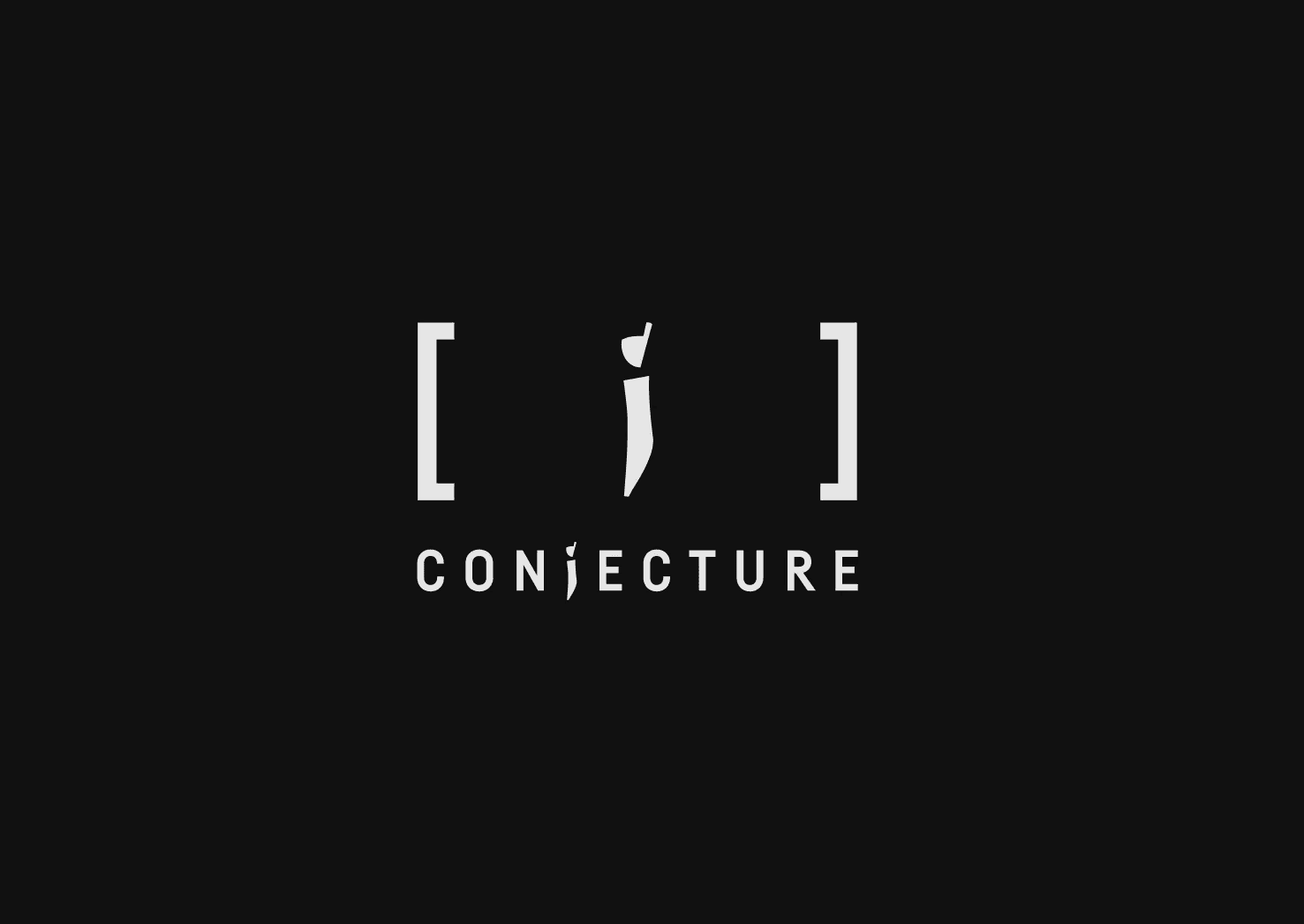
Feb 15, 2024
Conjecture: 2 Years
Conjecture: 2 Years
It has been 2 years since a group of hackers and idealists from across the globe gathered into a tiny, oxygen-deprived coworking space in downtown London with one goal in mind: Make the future go well, for everybody. And so, Conjecture was born.
Sign up to receive our newsletter and
updates on products and services.
Sign up to receive our newsletter and updates on products and services.
Sign up to receive our newsletter and updates on products and services.
Sign Up